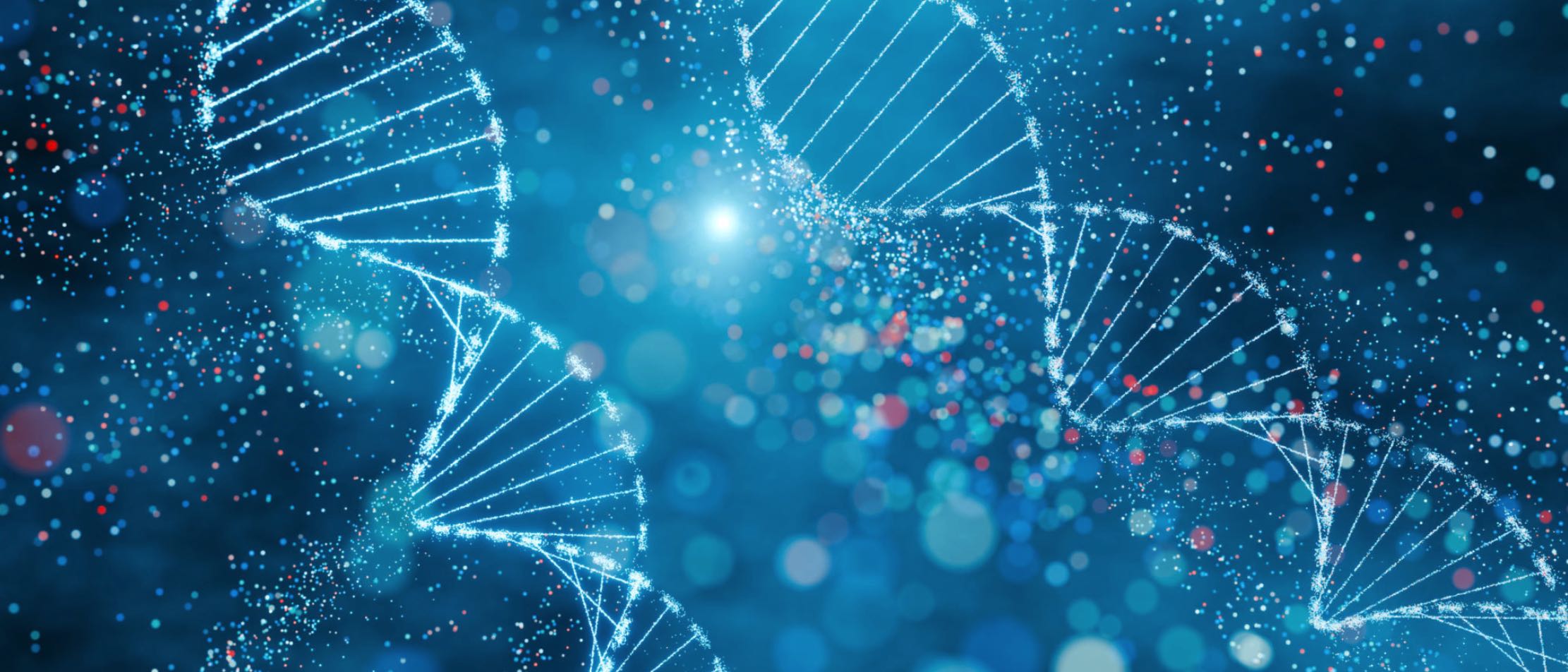
Structural Bioinformatics: Optimizing Lead Compounds for Targeted Therapies
Chapter 1: Introduction to Structural Bioinformatics
Overview of Bioinformatics in Drug Discovery
The integration of bioinformatics into drug discovery represents a transformative approach that enhances the efficiency and accuracy of identifying and developing new therapeutic agents. Bioinformatics encompasses a variety of computational tools and techniques that analyze biological data, particularly in genomics, proteomics, and metabolomics, to derive meaningful insights for drug development. This subchapter aims to provide an overview of how bioinformatics technologies facilitate various stages of drug discovery, from the identification of drug targets to the optimization of lead compounds and the assessment of drug efficacy.
Genomic data analysis is pivotal in drug target identification, allowing researchers to pinpoint specific molecular targets associated with diseases. High-throughput sequencing technologies generate extensive genomic datasets, which can be analyzed to identify mutations, gene expression levels, and pathways relevant to disease mechanisms. By applying bioinformatics tools to these datasets, scientists can uncover novel biomarkers and potential targets for drug intervention, enabling the development of therapies that are more specifically tailored to the molecular characteristics of diseases. This targeted approach not only increases the likelihood of therapeutic success but also helps in minimizing adverse effects by focusing on relevant biological pathways.
In addition to genomics, proteomics plays a crucial role in drug discovery by providing insights into protein interactions and functions. The application of proteomic techniques allows researchers to analyze the dynamic interactions between proteins within biological systems, elucidating how these interactions contribute to disease pathology. Bioinformatics tools enable the integration and interpretation of proteomic data, facilitating the identification of key proteins involved in disease processes that may serve as valuable drug targets. Understanding these interactions can inform the design of drugs that modulate specific protein functions, thereby enhancing therapeutic efficacy.
Metabolomics, the study of small molecules produced during metabolism, offers a complementary perspective in assessing drug efficacy and safety. By profiling metabolites in biological samples, researchers can gain insights into the biochemical effects of drug candidates on cellular pathways. Bioinformatics approaches are essential for processing and analyzing metabolomic data, allowing for the identification of metabolite changes associated with drug treatment. This information can inform decisions regarding the safety and effectiveness of new drug candidates, ultimately guiding the selection of compounds for further development.
Furthermore, the application of machine learning models in drug design represents a significant advancement in bioinformatics. These algorithms can analyze vast amounts of biological data to predict the effectiveness of new drug molecules based on established patterns and correlations. Machine learning techniques enhance the predictive power of drug discovery processes, enabling scientists to prioritize compounds with higher probabilities of success. When combined with structural bioinformatics, these approaches can further refine lead compounds by optimizing binding affinity and specificity, paving the way for the development of personalized medicine strategies that align with individual genetic profiles. Overall, the integration of bioinformatics in drug discovery not only streamlines the process but also fosters innovation, ultimately leading to more effective and tailored therapeutic options.
Importance of Structural Data in Lead Optimization
The optimization of lead compounds is a critical phase in drug development, and structural data plays a pivotal role in this process. Structural bioinformatics provides essential insights into the three-dimensional arrangements of molecules, allowing scientists to understand how lead compounds interact with their biological targets at the atomic level. This understanding is crucial for refining the binding affinity and specificity of these compounds, ultimately leading to more effective therapeutic agents. By leveraging structural data, researchers can make informed modifications to lead compounds, enhancing their potential to address specific diseases.
In the context of drug target identification, genomic data analysis is invaluable. By analyzing the genetic underpinnings of various diseases, researchers can identify potential drug targets and their associated pathways. Structural bioinformatics complements this by providing a detailed view of the target's molecular structure, aiding in the design of molecules that can effectively modulate these targets. This integration of genomic data with structural insights accelerates the identification of viable drug candidates, as scientists can prioritize targets based on their structural characteristics and biological relevance.
Proteomics further enhances our understanding of drug interactions by elucidating the complex relationships between proteins and their ligands. Structural data derived from proteomic analyses allows researchers to visualize protein structures and their conformational changes upon ligand binding. This information is critical for optimizing lead compounds, as it informs the design of molecules that can achieve desired interactions with targeted proteins. By incorporating proteomic insights into structural bioinformatics, scientists can improve the specificity and efficacy of lead compounds, leading to better therapeutic outcomes.
Metabolomics also plays a significant role in assessing the efficacy of new drug candidates. By analyzing the metabolic profiles of organisms in response to drug treatment, researchers can gain insights into how lead compounds influence biological systems. Structural data can guide the interpretation of metabolomic results, linking observed changes in metabolite levels to specific interactions at the molecular level. This holistic approach enables scientists to evaluate not only the effectiveness of lead compounds but also their safety profiles, ensuring that new therapies are both potent and tolerable.
Finally, the advent of machine learning models in drug design has opened new avenues for optimizing lead compounds using structural data. By applying advanced algorithms to vast datasets, researchers can predict the effectiveness of new drug molecules based on their structural features and biological activity. This predictive capability, when combined with structural insights, allows for a more streamlined and efficient optimization process. As drug discovery continues to evolve, the integration of structural bioinformatics with emerging technologies will be crucial in developing targeted therapies that are both innovative and effective.
Goals and Scope of the Book
The subchapter titled "Goals and Scope of the Book" outlines the key objectives and thematic focus of "Structural Bioinformatics: Optimizing Lead Compounds for Targeted Therapies." This book aims to bridge the gap between traditional drug discovery processes and modern computational techniques, thereby enhancing our understanding of how structural bioinformatics can be employed to optimize lead compounds for targeted therapies. The primary goal is to provide a comprehensive framework that integrates various bioinformatics technologies to facilitate new drug molecule discovery and development.
One significant area of focus is genomic data analysis for drug target identification. The book emphasizes the importance of leveraging genomic data to uncover potential drug targets for a range of diseases. By employing advanced bioinformatics approaches to analyze genetic information, scientists can identify specific molecular targets that are crucial for disease progression. This foundation sets the stage for the subsequent chapters, which explore how these identified targets can be effectively manipulated through drug design and development.
Proteomics also plays a critical role in the drug discovery process, and this book delves into the application of proteomic techniques to understand protein interactions. By examining how proteins interact within biological systems, researchers can gain insights into the mechanisms of drug action and resistance. This understanding is vital for the development of more effective therapies, as it allows for the identification of key protein targets that can be modulated by new drug candidates.
In addition to genomics and proteomics, the book addresses the emerging field of metabolomics for drug efficacy assessment. Utilizing metabolomic profiling enables scientists to evaluate the safety and effectiveness of new drug candidates by analyzing metabolic changes in response to treatment. This approach not only enhances drug development but also contributes to a more comprehensive understanding of how drugs exert their effects at the biochemical level.
The incorporation of machine learning models in drug design is another pivotal aspect of the book. By developing and applying machine learning algorithms, scientists can predict the effectiveness of new drug molecules based on extensive biological data. This predictive capability serves to streamline the drug discovery process, making it more efficient and targeted. The book also highlights the significance of virtual screening techniques for compound library exploration, enabling rapid assessment of large libraries to identify promising drug candidates. Together, these elements underscore the book's overarching goal: to provide a robust resource that empowers scientists to utilize structural bioinformatics in the optimization of lead compounds for targeted therapies.
Chapter 2: Genomic Data Analysis for Drug Target Identification
Introduction to Genomic Data
Genomic data has emerged as a cornerstone in the field of bioinformatics, particularly in the context of drug discovery and development. This subchapter introduces the multifaceted nature of genomic data, emphasizing its critical role in identifying potential drug targets for a variety of diseases. As the foundation for understanding genetic underpinnings, genomic data enables researchers to pinpoint alterations in genes, transcripts, and other genomic elements that contribute to disease pathogenesis. By harnessing this wealth of information, scientists can develop targeted therapies aimed at specific molecular targets, enhancing both efficacy and safety in drug development.
The advent of high-throughput sequencing technologies has revolutionized the acquisition of genomic data, allowing for comprehensive analyses of entire genomes, exomes, and transcriptomes. These technologies enable the identification of genetic variations, such as single nucleotide polymorphisms (SNPs) and copy number variations (CNVs), which can have significant implications for therapeutic interventions. By analyzing such variations across different populations and disease states, researchers can uncover novel biomarkers and therapeutic targets. This genomic insight is essential for the advancement of precision medicine, where treatments can be tailored to an individual’s unique genetic profile, thereby improving therapeutic outcomes.
In addition to identifying potential drug targets, genomic data plays a crucial role in the integration of multi-omics approaches, including proteomics and metabolomics. The relationship between genomic alterations and their downstream effects on protein expression and metabolite profiles is complex yet vital for understanding the mechanisms of drug action. By leveraging genomic data alongside proteomic techniques, scientists can elucidate protein interactions and signaling pathways that are critical for disease progression. This integrative approach not only enhances the understanding of biological systems but also aids in the identification of novel therapeutic candidates that may have been overlooked in traditional drug discovery paradigms.
Machine learning algorithms have become increasingly important in the analysis of genomic data, facilitating the prediction of drug efficacy and safety profiles based on biological information. By training models on large datasets, researchers can identify patterns and correlations that inform the design of new drug molecules. This application of artificial intelligence is particularly valuable in reducing the time and cost associated with drug development, as it enables the rapid screening of potential candidates before they enter the costly phases of clinical trials. The integration of machine learning with genomic data analysis represents a significant advancement in the field, providing a robust framework for the rational design of therapeutics.
In summary, the exploration of genomic data is integral to modern drug discovery and development. By understanding the genetic basis of diseases and leveraging advanced bioinformatics tools, scientists can identify and optimize drug targets, leading to the development of more effective and personalized therapies. As research continues to evolve, the interplay between genomic data analysis, proteomics, and machine learning will undoubtedly drive innovation, ultimately transforming the landscape of drug discovery and paving the way for groundbreaking therapeutic interventions.
Techniques for Analyzing Genomic Data
Genomic data analysis has become an essential aspect of drug discovery, particularly in identifying potential drug targets for various diseases. With the advent of high-throughput sequencing technologies, researchers can now generate vast amounts of genomic information, providing insights into the genetic underpinnings of diseases. Techniques such as differential gene expression analysis, genome-wide association studies (GWAS), and next-generation sequencing (NGS) allow scientists to pinpoint mutations or expression changes associated with specific pathologies. By correlating these genomic alterations with disease phenotypes, researchers can identify novel targets for therapeutic intervention, facilitating the development of more effective drugs.
Moreover, integrating genomic data with proteomics and metabolomics enhances our understanding of biological processes at multiple levels. While genomic data provides insights into the genetic basis of diseases, proteomics reveals the functional consequences of those genetic alterations. Techniques such as mass spectrometry and protein interaction assays help characterize the protein landscape affected by specific genomic events. By employing systems biology approaches, researchers can create comprehensive models that incorporate genomic, proteomic, and metabolomic data, allowing for a holistic view of disease mechanisms. This integration not only aids in target identification but also informs the design of drugs that can specifically modulate these targets.
Machine learning has emerged as a powerful tool for analyzing genomic data, particularly in predicting drug efficacy and safety profiles. Algorithms can be trained on large datasets to identify patterns and relationships within the data that might not be evident through traditional analytical methods. For instance, predictive models can assess the likelihood of a drug candidate's success based on genomic variations present in the target population. Such machine learning applications streamline the drug discovery process, enabling researchers to prioritize compounds for further investigation and reduce the time and cost associated with traditional experimental approaches.
In addition to identifying potential targets, genomic data analysis plays a crucial role in the optimization of lead compounds. Structural bioinformatics techniques, including molecular docking and simulations, allow scientists to explore the interactions between drug candidates and their biological targets. By leveraging genomic insights, researchers can refine lead compounds to enhance binding affinity and specificity. This iterative process of design and testing not only improves the potential success rate of drug candidates but also contributes to the development of personalized medicine approaches, where treatments can be tailored to the genetic profiles of individual patients.
Lastly, the integration of clinical data with genomic analyses further enhances drug development efforts. By analyzing real-world patient data alongside genetic information, researchers can better understand how genetic variations influence drug responses. This information is invaluable for drug repurposing strategies, where existing drugs are evaluated for new therapeutic indications based on their genomic activity profiles. As the field of bioinformatics continues to evolve, the techniques for analyzing genomic data will become increasingly sophisticated, paving the way for innovative therapeutic strategies that leverage genetic insights to improve patient outcomes.
Identifying Disease-Specific Drug Targets
Identifying disease-specific drug targets is a critical step in the drug discovery process, as it lays the foundation for developing effective therapies tailored to individual diseases. Advances in genomic data analysis have revolutionized our understanding of the molecular underpinnings of various diseases, facilitating the identification of potential drug targets. By examining genetic variations associated with diseases, researchers can pinpoint specific genes and their corresponding proteins that play pivotal roles in disease pathology. This genomic approach allows for the identification of novel targets that were previously unrecognized, thereby expanding the arsenal of potential therapeutic interventions.
Proteomics complements genomic data analysis by providing insights into the dynamic interactions of proteins within biological systems. By employing advanced proteomic techniques, scientists can study protein expression levels, modifications, and interactions that occur in disease states. This information is invaluable for understanding the functional implications of identified drug targets. For instance, proteomic profiling can reveal how certain proteins interact within signaling pathways, identifying key nodes that may be amenable to therapeutic intervention. Understanding these interactions is crucial for designing drugs that can effectively modulate disease-specific pathways.
Metabolomics also plays a significant role in the drug discovery process by assessing the efficacy and safety of new drug candidates. By analyzing the metabolite profiles of biological samples, researchers can gain insights into the biochemical changes induced by drug treatments. This approach not only aids in evaluating the pharmacodynamics of new drug molecules but also helps in identifying biomarkers of drug response. Such biomarkers can guide clinical decisions, ensuring that therapies are both effective and safe for patients. The integration of metabolomic data into the drug discovery workflow enhances our ability to make informed decisions regarding the viability of drug candidates.
The application of machine learning models in drug design has further transformed the identification of disease-specific drug targets. By leveraging large datasets from genomic, proteomic, and metabolomic studies, machine learning algorithms can uncover complex patterns and relationships that may not be immediately apparent through traditional analytical methods. These models can predict the efficacy of new drug molecules by analyzing biological data, streamlining the process of drug candidate selection. Additionally, machine learning can assist in optimizing lead compounds by predicting their binding affinities and specificity toward identified targets, thereby enhancing the chances of successful therapeutic outcomes.
Finally, systems biology approaches integrate various biological data to provide a holistic view of disease mechanisms, which is essential for drug target identification. By modeling the intricate interactions within cellular networks, researchers can identify potential therapeutic targets that may influence multiple pathways. This comprehensive approach not only enhances our understanding of disease biology but also aids in the rational design of drugs that can address the complexity of diseases. The integration of bioinformatics tools across these various domains creates a robust framework for the identification and validation of disease-specific drug targets, ultimately leading to the development of more effective and personalized therapies.
Case Studies: Successful Target Identification
Case studies in successful target identification illustrate the transformative potential of integrating genomic data, proteomics, and machine learning in drug discovery. The case of trastuzumab, an antibody targeting the HER2 receptor in breast cancer, exemplifies the power of genomic analysis in identifying therapeutic targets. Initially, the overexpression of the HER2 gene was discovered through genomic profiling, leading to the development of trastuzumab. This targeted therapy not only improved patient outcomes but also set a precedent for the application of genomics in personalizing treatment strategies for cancer patients, highlighting how genomic data can inform drug target identification and validate therapeutic approaches.
In another compelling instance, the use of proteomics has significantly advanced our understanding of complex protein interactions and their roles in disease. The identification of the BRAF V600E mutation as a target in melanoma demonstrates the effectiveness of proteomic techniques in drug discovery. Through mass spectrometry and other proteomic technologies, researchers were able to characterize the altered protein and its signaling pathways, leading to the development of BRAF inhibitors. This case underscores the importance of proteomics in elucidating the molecular underpinnings of diseases, paving the way for targeted therapies that directly address the specific alterations in protein function.
Metabolomics has also emerged as a vital tool in evaluating the efficacy and safety of new drug candidates. The success of the anti-diabetic drug metformin exemplifies how metabolomic profiling can inform drug development. Researchers used metabolomic techniques to analyze metabolic pathways affected by the drug, leading to insights into its mechanism of action and potential side effects. Such assessments not only aid in the selection of candidates for further development but also contribute to a deeper understanding of the drug’s impact on metabolic networks, ensuring that new therapies are both effective and safe for patients.
The incorporation of machine learning models into drug design has revolutionized the way researchers approach target identification and lead optimization. A notable example is the use of deep learning algorithms to predict the efficacy of novel compounds based on biological data. By analyzing large datasets of chemical structures and biological activity, researchers can identify promising drug candidates with higher accuracy and efficiency than traditional methods. This case exemplifies the growing importance of artificial intelligence in bioinformatics, showcasing how machine learning can streamline the discovery process and enhance the likelihood of clinical success.
Lastly, the integration of systems biology approaches in pharmacology has provided a comprehensive framework for understanding the complex interactions within biological systems during drug discovery. A case study involving the development of combination therapies for HIV illustrates how systems biology can inform drug target identification and optimize treatment regimens. By modeling the interactions between various biological components, researchers can identify synergistic effects among drugs, leading to more effective and personalized therapies. This holistic approach not only enhances our understanding of disease mechanisms but also facilitates the identification of novel targets that might have been overlooked through traditional methods.
Chapter 3: Proteomics in Drug Discovery
Fundamentals of Proteomics
The fundamentals of proteomics play a crucial role in understanding the molecular underpinnings of diseases and the development of targeted therapies. Proteomics, the large-scale study of proteins, particularly their functions and structures, enables researchers to analyze the dynamic protein composition of a cell, tissue, or organism at a specific point in time. This discipline is essential in drug discovery, as proteins are often the primary targets of therapeutic agents. By employing advanced techniques such as mass spectrometry and two-dimensional gel electrophoresis, scientists can identify protein expressions, modifications, and interactions that are pivotal for elucidating disease mechanisms and discovering new drug targets.
Understanding the interplay between genomic data and proteomic profiles is vital for drug target identification. Genomic data provides a blueprint of potential therapeutic targets by revealing the genetic mutations and variations associated with specific diseases. However, the ultimate expression and functionality of these targets can only be comprehensively understood through proteomic analysis. By integrating genomic and proteomic data, researchers can prioritize targets based on their biological relevance and potential for therapeutic intervention. This holistic approach enhances the precision of drug development efforts and facilitates the discovery of novel targets that may have been overlooked when considering genomic data alone.
Proteomics is also instrumental in dissecting protein interactions, which are critical for drug discovery. The identification of protein-protein interactions and their functional consequences can reveal pathways that are altered in disease states, illuminating potential intervention points for new drugs. Techniques such as affinity purification coupled with mass spectrometry allow for the comprehensive mapping of interaction networks, providing insights into the cellular context of protein functions. Understanding these interactions is essential not only for the identification of new drug targets but also for designing compounds that can modulate these interactions effectively.
Moreover, proteomic techniques can be used to assess drug efficacy and safety through metabolomics, which focuses on the small molecules resulting from metabolic processes. By profiling the metabolites in response to drug treatment, researchers can gain insights into the biochemical pathways affected by a drug. This information is invaluable for evaluating the therapeutic potential and potential side effects of new drug candidates. The integration of proteomics and metabolomics provides a systems-level understanding of how drugs influence biological systems, paving the way for the development of safer and more effective therapies.
In conclusion, the fundamentals of proteomics are integral to advancing drug discovery and development. By leveraging proteomic data, scientists can identify and validate drug targets, understand protein interactions, and assess the efficacy of new drug candidates. As the field of bioinformatics continues to evolve, the integration of proteomics with genomic, metabolomic, and clinical data will enhance our understanding of complex biological systems and support the design of personalized therapeutic strategies. Ultimately, this multifaceted approach will lead to the development of more effective and tailored treatments for a variety of diseases, transforming the landscape of modern medicine.
Techniques and Technologies in Proteomics
In the rapidly evolving field of drug discovery, proteomics plays a crucial role in understanding the complex interactions between proteins and their impact on biological processes. Techniques and technologies in proteomics have advanced significantly, enabling researchers to analyze protein expression, modification, and interactions at an unprecedented scale. Key methods such as mass spectrometry (MS) and two-dimensional gel electrophoresis (2DE) are central to proteomic studies. Mass spectrometry, in particular, has become a standard tool for identifying and quantifying proteins in complex biological samples, allowing scientists to generate comprehensive proteomic profiles that can reveal potential drug targets and biomarkers for various diseases.
Liquid chromatography coupled with mass spectrometry (LC-MS) is one of the most powerful techniques in proteomics, facilitating the separation and analysis of proteins in a mixture. This method enhances sensitivity and specificity, enabling the detection of low-abundance proteins that may play critical roles in disease mechanisms. Additionally, advancements in high-resolution mass spectrometry have improved the accuracy and reliability of protein identification and quantification. Coupled with bioinformatics tools for data analysis, LC-MS provides a robust platform for exploring protein interactions and post-translational modifications, which are essential for understanding the functional implications of proteins in drug development.
Another key technology in proteomics is protein microarrays, which enable the simultaneous analysis of thousands of proteins in a high-throughput format. These arrays can be used to study protein-protein interactions, enzyme activity, and antibody specificity, making them invaluable for identifying novel drug targets. The ability to profile proteins in response to various drug candidates allows researchers to assess the efficacy of compounds and understand their mechanisms of action. This high-throughput approach accelerates the drug discovery process by providing comprehensive insights into the proteome and its dynamic changes in disease states.
Integrating proteomics with genomics enhances the identification of drug targets and therapeutic strategies. The combination of genomic data with proteomic analysis allows for a systems biology approach, providing a holistic view of biological pathways and processes. By correlating genomic alterations with protein expression profiles, researchers can identify potential drug targets that are not only relevant to the disease but also amenable to therapeutic intervention. This integration is particularly important in cancer research, where understanding the proteomic landscape can inform personalized medicine strategies aimed at tailoring treatments to individual patients based on their unique molecular profiles.
The application of machine learning algorithms in proteomics further enhances the ability to predict protein functions and interactions, leading to more effective drug design. By leveraging large datasets generated from proteomic studies, machine learning models can identify patterns and correlations that traditional methods might overlook. This predictive capability is crucial for optimizing lead compounds and assessing their potential efficacy and safety before entering clinical trials. As researchers continue to refine these techniques, proteomics will remain a cornerstone of drug discovery, paving the way for innovative therapies that are based on a deep understanding of the molecular underpinnings of disease.
Understanding Protein Interactions
Understanding protein interactions is crucial in the realm of structural bioinformatics, especially when optimizing lead compounds for targeted therapies. Proteins, as fundamental building blocks of cellular processes, engage in a myriad of interactions that dictate their functionality. These interactions occur at various levels, from simple binding events between proteins and ligands to complex networks that involve multiple proteins and cellular components. By elucidating these interactions, scientists can identify potential drug targets and understand the mechanisms underlying various diseases, thereby enhancing the drug discovery process.
In the context of genomic data analysis, understanding protein interactions plays a vital role in drug target identification. Genomic sequences can provide insights into the presence of specific genes and their encoded proteins, which can subsequently reveal potential therapeutic targets. By employing bioinformatics tools to analyze genomic data, researchers can identify proteins that are overexpressed or mutated in disease states. These proteins often interact with other cellular molecules, forming pathways that can be disrupted by new drug candidates. Thus, a thorough understanding of these interactions allows for a more informed selection of drug targets that are likely to yield effective therapeutic outcomes.
Proteomics, the large-scale study of proteins, further deepens our understanding of protein interactions and their implications in drug development. Advanced proteomic techniques, such as mass spectrometry and protein microarrays, enable the identification of protein-protein interactions and post-translational modifications that influence protein function. By mapping these interactions, researchers can gain insights into the signaling pathways affected by disease, allowing for the identification of novel drug targets. Moreover, characterizing these interactions can reveal potential off-target effects of drug candidates, facilitating the design of compounds with greater specificity and reduced side effects.
Metabolomics complements the study of protein interactions by providing a snapshot of the biochemical state of a cell or organism. By profiling metabolites, researchers can assess the efficacy and safety of new drug candidates in real time. The interplay between proteins and metabolites is critical; proteins often serve as enzymes that catalyze metabolic reactions, and their interactions with small molecules can influence metabolic pathways. Understanding these relationships can help in evaluating how drugs affect cellular metabolism and, ultimately, therapeutic outcomes. Thus, integrating metabolomic data with protein interaction studies enhances the overall understanding of drug efficacy.
The advent of machine learning models has transformed the landscape of drug design, particularly in predicting protein interactions based on biological data. By training algorithms on large datasets of known protein interactions, these models can identify patterns and make predictions about novel interactions. This capability is particularly valuable in virtual screening processes, where large compound libraries are evaluated for potential drug candidates. By integrating machine learning with structural bioinformatics, scientists can refine and optimize lead compounds, ensuring that they exhibit high binding affinity and specificity towards their intended targets. Understanding protein interactions, therefore, is not just a foundational aspect of drug discovery, but a dynamic field that continues to evolve with technological advancements.
Implications of Proteomics in Drug Development
The implications of proteomics in drug development are profound, as this field provides critical insights into the complex interactions and functions of proteins within biological systems. Proteomics, the large-scale study of proteins, is essential for understanding the molecular mechanisms that underlie diseases. By identifying and characterizing proteins involved in disease pathways, researchers can pinpoint potential drug targets with greater precision. This capability is particularly important in the context of personalized medicine, where tailoring drug therapies to an individual's unique protein expression profile can enhance therapeutic efficacy and reduce adverse effects.
Recent advancements in proteomic technologies, including mass spectrometry and protein microarrays, have revolutionized the way scientists approach drug discovery. These techniques enable the comprehensive analysis of protein expression levels, post-translational modifications, and protein-protein interactions. By integrating proteomic data with genomic and metabolomic information, researchers can construct a more holistic view of disease mechanisms. This systems biology approach not only aids in the identification of novel drug targets but also facilitates the understanding of drug action and resistance mechanisms, which are critical for developing effective therapies.
Moreover, proteomics plays a pivotal role in assessing drug efficacy and safety. By utilizing proteomic profiling, scientists can evaluate how drug candidates affect the proteome of target cells or tissues, providing insights into their pharmacodynamics and potential off-target effects. This information is invaluable in early-phase drug development, as it allows researchers to make informed decisions about which candidates to advance based on robust biological data. Additionally, understanding the proteomic changes induced by drug treatment can help identify biomarkers for patient stratification, ultimately leading to more effective and personalized therapeutic strategies.
The integration of machine learning models with proteomic data further enhances drug discovery efforts. By leveraging large datasets derived from proteomic studies, machine learning algorithms can identify patterns and predict the interactions between drugs and their protein targets. This predictive capability accelerates the lead optimization process by allowing scientists to focus on compounds that are more likely to succeed in clinical trials. Furthermore, machine learning approaches can assist in virtual screening of compound libraries, identifying synergistic effects between drugs and their targets, and offering insights into potential drug repurposing opportunities.
In summary, the implications of proteomics in drug development extend beyond target identification to encompass the entire drug discovery pipeline. By providing a detailed understanding of protein dynamics and interactions, proteomics empowers researchers to design more effective and safer therapeutics. As this field continues to evolve, its integration with bioinformatics, machine learning, and other technologies will undoubtedly lead to innovative approaches in drug development, ultimately enhancing the precision and efficacy of therapies for a wide range of diseases.
Chapter 4: Metabolomics for Drug Efficacy Assessment
Introduction to Metabolomics
Metabolomics, the comprehensive study of small molecules or metabolites within biological systems, has emerged as a pivotal field in the landscape of drug discovery and development. This subchapter aims to provide an overview of metabolomics, elucidating its significance in understanding biological processes and its applications in evaluating drug efficacy and safety. As the complexity of biological systems becomes increasingly apparent, metabolomics offers a unique lens through which scientists can profile metabolic alterations associated with disease states and therapeutic interventions.
In drug discovery, the integration of metabolomics with other omics technologies, such as genomics and proteomics, enhances the identification of drug targets and the evaluation of drug candidates. By examining metabolic profiles, researchers can uncover biomarkers that indicate disease progression or response to treatment, thereby enabling more informed decisions in drug development. This holistic approach facilitates the identification of potential drug targets that may not be evident through genomic or proteomic analyses alone, thus broadening the scope of therapeutic strategies.
The application of metabolomics in assessing drug efficacy is particularly noteworthy. Metabolomic profiling allows for the monitoring of metabolic changes in response to drug treatment, providing insights into the pharmacodynamics and pharmacokinetics of new drug candidates. By comparing the metabolic signatures of treated versus untreated biological samples, scientists can gauge the effectiveness of a drug and its potential side effects. This capability is crucial for optimizing lead compounds, as it helps to determine which candidates warrant further investigation based on their impact on metabolic pathways.
Furthermore, the integration of machine learning models with metabolomic data offers exciting prospects for drug design. By employing advanced computational techniques, researchers can analyze complex metabolic data sets to predict drug responses and optimize therapeutic strategies. Machine learning algorithms can identify patterns in metabolomic profiles that correlate with drug efficacy or toxicity, thereby enhancing the decision-making process in lead compound optimization. This intersection of technology and biological data underscores the transformative potential of metabolomics in modern pharmacology.
In summary, metabolomics serves as a critical tool in the quest for new therapeutics, bridging the gap between molecular biology and clinical application. Its ability to provide a detailed understanding of metabolic changes in response to drug treatment contributes significantly to the development of targeted therapies. As the field of bioinformatics continues to evolve, the integration of metabolomics with other omics technologies and advanced computational methods will play an essential role in shaping the future of drug discovery and personalized medicine. This subchapter sets the stage for exploring these synergies and their implications for optimizing lead compounds in targeted therapies.
Profiling Metabolites for Drug Evaluation
Profiling metabolites plays a crucial role in drug evaluation, particularly in understanding how new drug candidates affect biological systems at a metabolic level. Metabolomics, the comprehensive study of metabolites within biological samples, allows researchers to capture the dynamic changes that occur in cellular metabolism in response to drug treatment. This subchapter explores the methodologies and implications of metabolite profiling for assessing the efficacy and safety of drug candidates, offering insights into how these approaches can be integrated into the drug development pipeline.
One of the primary advantages of metabolite profiling is its ability to provide a holistic view of the biochemical alterations induced by drug exposure. By employing techniques such as mass spectrometry (MS) and nuclear magnetic resonance (NMR) spectroscopy, scientists can obtain detailed information about metabolic pathways that are influenced by a drug. This information is invaluable for identifying biomarkers of drug efficacy and toxicity, which can aid in the selection of lead compounds with the most favorable profiles. Moreover, the identification of specific metabolic signatures associated with therapeutic responses can facilitate the stratification of patients in clinical trials, ultimately leading to more personalized treatment approaches.
In addition to evaluating drug efficacy, metabolomics can also contribute significantly to safety assessments. By monitoring changes in metabolite levels, researchers can detect potential adverse effects that may not be evident through traditional pharmacological evaluations. Understanding the toxicological impact of a drug at the metabolic level can help in identifying off-target effects and potential drug-drug interactions. This is particularly important in the context of polypharmacy, where patients may be taking multiple medications simultaneously. Profiling metabolites provides a means to comprehensively assess how these various compounds interact within the biological system.
The integration of metabolomic data with other omics technologies, such as genomics and proteomics, enhances the overall understanding of drug mechanisms. For example, by correlating metabolic changes with genomic data, researchers can identify genetic variants that may influence metabolic responses to a drug. Similarly, proteomic analyses that elucidate protein interactions and pathways can complement metabolomic findings, creating a more complete picture of how a drug exerts its effects. This systems biology approach not only aids in identifying potential drug targets but also enhances the predictive power of machine learning models used in drug design.
Finally, the utility of metabolite profiling extends to the optimization of lead compounds. By analyzing the metabolic consequences of drug candidates, researchers can refine their structures to enhance desired effects while minimizing adverse outcomes. Through iterative cycles of design, testing, and evaluation, metabolomic profiling serves as a crucial feedback mechanism in the lead optimization process. As drug discovery increasingly relies on data-driven approaches, the incorporation of metabolomic insights will be essential for developing safe and effective therapies that meet the complex needs of diverse patient populations.
Assessing Safety and Efficacy through Metabolomic Data
Assessing safety and efficacy through metabolomic data represents a pivotal advancement in the landscape of drug discovery and development. Metabolomics, the comprehensive study of metabolites within biological systems, offers a unique window into the biochemical state of an organism, enabling scientists to evaluate the biochemical impacts of drug candidates. By profiling metabolites before and after drug administration, researchers can uncover crucial insights regarding the drug's mechanism of action, potential side effects, and overall metabolic changes induced by the treatment. This approach not only aids in understanding the therapeutic potential of new compounds but also in identifying biomarkers for safety and efficacy.
The integration of metabolomic data into drug development processes aligns seamlessly with the goals of systems biology. By examining the dynamic interactions between drugs and metabolic pathways, scientists can gain a holistic view of a drug’s impact on biological systems. This systems-level understanding is essential in identifying off-target effects and potential toxicities that may not be apparent through traditional pharmacological assessments. Therefore, leveraging metabolomics in conjunction with genomic and proteomic data can enhance the predictive accuracy of drug efficacy and safety profiles, thus supporting the design of safer therapeutic agents.
To optimize lead compounds effectively, it is imperative to analyze how metabolic changes correlate with therapeutic outcomes. Metabolomic profiling can identify specific metabolic signatures associated with effective treatment responses, allowing researchers to delineate which drug candidates are likely to elicit the desired pharmacological effects. Such insights can inform lead optimization efforts, guiding structural modifications to enhance drug performance while minimizing adverse effects. By employing machine learning models trained on metabolomic data, scientists can predict the likelihood of success for newly designed compounds, streamlining the drug development pipeline.
Moreover, the application of metabolomics extends beyond initial drug candidate evaluation to encompass understanding drug repurposing opportunities. Existing medications can be re-evaluated using metabolomic data to uncover new therapeutic uses based on their metabolic impact. This approach could significantly expedite the drug discovery process by identifying novel applications for compounds that have already undergone extensive safety evaluations. As the field of bioinformatics continues to evolve, the integration of metabolomic analyses with computational tools will further enhance the capability to repurpose drugs effectively.
In conclusion, assessing safety and efficacy through metabolomic data is an essential component of modern drug discovery and development. This multifaceted approach not only facilitates the identification of promising drug candidates but also augments the understanding of their pharmacodynamics and safety profiles. By embracing the insights provided by metabolomics, along with genomic, proteomic, and machine learning methodologies, scientists can significantly improve the precision of drug design and optimization, ultimately leading to more effective and safer therapeutic interventions.
Case Studies: Metabolomics in Action
Metabolomics, the comprehensive study of metabolites within biological systems, has emerged as a pivotal tool in drug discovery and development. This subchapter explores various case studies that illustrate how metabolomics is applied to assess drug efficacy, identify potential therapeutic targets, and understand the biochemical landscapes associated with different diseases. By examining specific examples, we can discern the transformative impact of metabolomics on optimizing lead compounds and guiding therapeutic strategies.
In one notable case study, researchers investigated the metabolic profiles of patients with type 2 diabetes to identify biomarkers that could predict therapeutic responses to specific drug treatments. Through a combination of mass spectrometry and nuclear magnetic resonance spectroscopy, the team analyzed serum samples from participants before and after treatment with a novel antidiabetic agent. The resulting metabolomic data revealed distinct patterns that correlated with improvements in glycemic control, thereby demonstrating the utility of metabolomics in evaluating drug efficacy. This approach not only provided insights into patient-specific responses but also highlighted the potential for tailoring therapies based on individual metabolic profiles.
Another compelling example involves the use of metabolomics in cancer therapy. A study focused on the metabolic changes in tumor cells subjected to a new chemotherapeutic agent revealed critical insights into the drug's mechanism of action. By employing targeted metabolomic analyses, researchers identified specific pathways that were upregulated in response to treatment, offering a clearer understanding of the drug's effects at the cellular level. This knowledge not only aids in assessing drug efficacy but also opens avenues for the development of combination therapies that exploit these metabolic vulnerabilities, thereby enhancing overall treatment outcomes.
The integration of metabolomics with other omics data, such as genomics and proteomics, further enhances its application in drug discovery. A case study examining metabolic alterations in patients with cardiovascular diseases combined genomic data to identify novel drug targets. By correlating metabolomic profiles with genetic variations, the researchers were able to pinpoint specific metabolic pathways that could be targeted for therapeutic intervention. This systems biology approach underscores the importance of a multi-omics framework in understanding complex disease mechanisms and optimizing drug development efforts.
Lastly, the advent of machine learning has revolutionized the analysis of metabolomic data, enabling the identification of predictive models for drug efficacy. In a recent study, machine learning algorithms were trained on extensive metabolomic datasets to predict the outcomes of clinical trials for a new antidepressant. The models successfully identified key metabolites associated with therapeutic success, illustrating the potential for machine learning to streamline the drug development process. By harnessing the power of metabolomics alongside advanced computational techniques, researchers can better inform decision-making in lead compound optimization and therapeutic strategy formulation.
Through these case studies, it is evident that metabolomics plays a crucial role in the drug discovery and development landscape. By providing insights into drug efficacy, identifying biomarkers, and facilitating the integration of multi-omics data, metabolomics not only enhances our understanding of biological systems but also paves the way for innovative therapeutic approaches tailored to individual patient needs.
Chapter 5: Machine Learning Models in Drug Design
Overview of Machine Learning in Drug Discovery
Machine learning (ML) has emerged as a transformative force in the field of drug discovery, significantly enhancing the efficiency and effectiveness of identifying new therapeutic candidates. By leveraging vast datasets from genomics, proteomics, metabolomics, and other biological domains, ML algorithms can uncover complex patterns and relationships that traditional methods may overlook. In the context of drug discovery, ML not only accelerates the identification of potential drug targets but also streamlines the optimization of lead compounds, ultimately leading to more personalized and effective treatment options.
The application of machine learning in drug discovery begins with genomic data analysis, where algorithms are employed to sift through massive datasets generated from high-throughput sequencing technologies. These analyses can identify genetic variants associated with diseases, enabling researchers to pinpoint potential drug targets. By utilizing supervised and unsupervised learning techniques, ML can help classify genes based on their roles in disease pathways, making it possible to prioritize targets for further investigation. This genomic focus is crucial for developing therapies that are tailored to specific patient populations, as it facilitates the identification of targets that are most likely to yield effective treatments.
Proteomics, the study of proteins and their functions, also benefits greatly from machine learning methodologies. Proteins are integral to virtually all biological processes, and understanding their interactions can reveal critical insights into disease mechanisms. Machine learning models can analyze complex proteomic data to predict protein-protein interactions, identify biomarkers of disease, and assess the impact of various compounds on protein function. This application not only aids in the identification of potential therapeutic targets but also enhances our understanding of how drugs can modulate biological pathways at the molecular level, thereby improving the design of new drug candidates.
In addition to genomic and proteomic analyses, metabolomics plays a vital role in assessing drug efficacy and safety. By profiling the metabolic changes associated with drug administration, machine learning algorithms can identify biomarkers indicative of a drug's pharmacological effects or potential adverse reactions. This approach allows researchers to evaluate the therapeutic window of new drug candidates more effectively and to optimize dosing regimens based on individual metabolic responses. The integration of metabolomic data with machine learning techniques thus provides a comprehensive framework for understanding the pharmacodynamics and pharmacokinetics of new therapies.
Finally, machine learning models are increasingly being used to predict the effectiveness of new drug molecules based on a variety of biological data inputs. By employing algorithms that learn from previous successes and failures in drug development, researchers can forecast the likelihood of a compound's success in clinical trials. This predictive capability not only enhances the efficiency of the drug development process but also reduces the associated costs and timelines, allowing for a more agile response to emerging health challenges. As machine learning continues to evolve, its integration into structural bioinformatics and systems biology will further optimize lead compound identification and development, paving the way for innovative and targeted therapies in modern medicine.
Algorithms and Techniques for Drug Design
The landscape of drug design has evolved significantly with the advent of advanced algorithms and techniques that harness the power of computational methods. In structural bioinformatics, these algorithms play a crucial role in optimizing lead compounds for targeted therapies. By integrating data from various biological sources, researchers can effectively identify potential drug candidates and refine them for better therapeutic efficacy. This subchapter delves into the multifaceted algorithms and techniques that underpin modern drug design, focusing on their applications across genomic data analysis, proteomics, metabolomics, and machine learning.
Genomic data analysis is a cornerstone of drug target identification, enabling scientists to pinpoint potential targets associated with various diseases. Algorithms that mine genomic datasets can uncover mutations, gene expression patterns, and other genetic markers that are indicative of disease states. Techniques such as genome-wide association studies (GWAS) and next-generation sequencing (NGS) facilitate the identification of novel targets for drug development. By correlating these genomic insights with known biological pathways, researchers can prioritize targets for therapeutic intervention, paving the way for precision medicine approaches that tailor treatments to individual patient profiles.
Proteomics further enriches the drug discovery process by providing insights into protein interactions and functions within biological systems. Techniques such as mass spectrometry and protein microarrays are employed to generate comprehensive protein interaction networks. Algorithms designed to analyze these complex datasets can help elucidate the roles of specific proteins in disease mechanisms, guiding the selection of targets for drug development. Moreover, understanding post-translational modifications and protein folding can highlight potential binding sites for lead compounds, thereby enhancing the rational design of drugs that specifically modulate protein activity.
Incorporating metabolomics into drug efficacy assessment offers another layer of depth to the drug design process. By profiling metabolites in biological samples, researchers can evaluate the pharmacokinetics and pharmacodynamics of new drug candidates. Algorithms that analyze metabolomic data can identify biomarkers of drug response, allowing for the assessment of efficacy and safety early in the drug development pipeline. This holistic approach ensures that only the most promising candidates progress through clinical trials, ultimately reducing the time and resources spent on ineffective compounds.
The integration of machine learning models in drug design has revolutionized the ability to predict the effectiveness of new drug molecules. These algorithms leverage large datasets from genomics, proteomics, and metabolomics to train models that can forecast binding affinities, toxicity, and overall drug behavior. Techniques such as cheminformatics and quantitative structure-activity relationship (QSAR) modeling enable researchers to virtually screen vast compound libraries, identifying candidates that are most likely to succeed in clinical applications. As drug discovery continues to evolve, the synergy between bioinformatics and machine learning will be pivotal in creating innovative therapies that address unmet medical needs efficiently.
Predicting Drug Efficacy Using Biological Data
Predicting drug efficacy using biological data is a critical endeavor in modern drug discovery and development. As scientists strive to design targeted therapies that are both effective and safe, the integration of various biological data types becomes essential. Genomic data analysis, proteomics, and metabolomics are three primary facets that provide insights into drug target identification, drug interactions, and overall drug efficacy. By leveraging these data sources, researchers can better understand the underlying mechanisms of diseases and tailor their drug development strategies accordingly.
Genomic data analysis plays a pivotal role in identifying potential drug targets for various diseases. By examining variations in genetic sequences, researchers can pinpoint mutations and other genomic alterations that contribute to disease pathogenesis. This information allows for the identification of novel therapeutic targets, which can be further validated through proteomic techniques. Proteomics enables scientists to investigate protein interactions and expression levels, providing a comprehensive view of the biological pathways involved in disease. Understanding these interactions is crucial for predicting how a drug will behave in a biological system and how it may interact with its intended target.
Metabolomics adds another layer to the assessment of drug efficacy by profiling the metabolic changes that occur in response to drug treatment. This approach allows researchers to evaluate the safety and effectiveness of new drug candidates by examining changes in metabolite levels associated with beneficial or adverse effects. By correlating metabolomic profiles with clinical outcomes, scientists can gather valuable insights into how a drug influences biological processes, leading to improved efficacy and reduced side effects. This data-driven approach enhances the predictive power of drug efficacy assessments, providing a more detailed understanding of a drug's impact at the molecular level.
Incorporating machine learning models into drug design further enhances the prediction of drug efficacy based on biological data. By training algorithms on large datasets of biological information, researchers can develop predictive models that assess the likelihood of a drug's success in clinical settings. These models can identify patterns and relationships within the data that may not be immediately apparent, allowing for a more informed decision-making process in drug development. Machine learning tools also facilitate the optimization of lead compounds, identifying modifications that may improve binding affinity and specificity for drug targets.
Finally, the integration of systems biology approaches into pharmacology allows for a holistic understanding of complex interactions within biological systems during drug discovery. By considering the interplay between various biological components, researchers can better predict how drugs will function in vivo. This systems-level perspective, combined with virtual screening techniques for compound exploration, bioinformatics tools for drug repurposing, and personalized medicine strategies, underscores the importance of biological data in predicting drug efficacy. As the field continues to evolve, the synergy of these approaches will enhance our ability to develop more effective, targeted therapies tailored to individual patient needs.
Future Trends in Machine Learning Applications
The future of machine learning (ML) applications in the realm of bioinformatics is poised to revolutionize drug discovery and development. As the volume and complexity of biological data continue to escalate, leveraging machine learning algorithms is becoming increasingly essential. These techniques facilitate the identification of novel drug targets through genomic data analysis, which allows researchers to sift through vast datasets to pinpoint genes and pathways associated with various diseases. As ML models evolve, their ability to unearth hidden patterns in genomic data will enhance the efficiency of drug target identification, ultimately leading to more precise therapeutic interventions.
In the context of proteomics, machine learning will play a pivotal role in understanding protein interactions that are crucial for drug development. As proteomic data becomes more accessible, the integration of ML techniques will enable scientists to predict how proteins interact within complex biological systems. This predictive capability can inform the design of drugs that specifically target these interactions, improving the likelihood of therapeutic success. Moreover, the application of advanced ML algorithms will allow for the analysis of proteomic profiles on a scale previously unattainable, offering insights that could lead to the discovery of new therapeutic targets and biomarkers.
Metabolomics is another area where machine learning is set to make significant strides. As researchers utilize metabolomic profiling to evaluate the efficacy and safety of new drug candidates, ML models will be critical in interpreting the intricate relationships between metabolites and drug responses. By correlating metabolomic data with biological outcomes, machine learning can help predict how a drug will behave in different biological contexts. This capability not only accelerates the drug development process but also enhances the understanding of drug mechanisms, ultimately leading to safer and more effective therapies.
The application of machine learning in structural bioinformatics is expected to refine lead compound optimization significantly. By employing ML algorithms to predict binding affinities and specificities, researchers can streamline the process of lead optimization, reducing the time and resources spent on less promising candidates. Furthermore, as virtual screening techniques become more sophisticated, ML can enhance the evaluation of large compound libraries, allowing scientists to identify potential drug candidates more efficiently. The integration of machine learning in these processes promises to shorten development timelines and increase the success rates of new drug molecules.
Lastly, as the field moves toward personalized medicine, machine learning will be instrumental in tailoring drug therapies based on individual genetic profiles. By analyzing patient-specific genomic and clinical data, ML models can identify the most effective treatment options for diverse patient populations. This shift towards personalized medicine underscores the importance of integrating clinical data with preclinical findings, enabling a more holistic approach to drug development. As machine learning continues to advance, its applications within bioinformatics will provide transformative insights that not only enhance our understanding of complex biological systems but also pave the way for innovative therapeutic strategies.
Chapter 6: Structural Bioinformatics for Lead Compound Optimization
Role of Structural Bioinformatics in Drug Design
Structural bioinformatics plays a pivotal role in the drug design process by providing insights into the molecular interactions between potential drug candidates and biological targets. This field integrates computational tools and techniques to analyze the three-dimensional structures of biomolecules, which is essential for understanding how drugs can modulate biological functions. By examining the conformation of proteins and their complexes with ligands, researchers can identify critical binding sites and predict the binding affinity of new compounds. This knowledge enables the optimization of lead compounds, ensuring that they fit snugly into their target sites, thereby enhancing their therapeutic potential.
One of the primary applications of structural bioinformatics in drug design is in the identification of drug targets through genomic data analysis. By leveraging genomic information, scientists can identify proteins that are implicated in specific disease pathways. Structural bioinformatics tools facilitate the modeling of these proteins, allowing researchers to visualize their structures and understand how mutations or variations can affect their function. This structural insight is crucial for designing drugs that can either restore normal function or inhibit aberrant activities associated with disease states.
Proteomics also greatly benefits from the integration of structural bioinformatics in drug discovery. By analyzing protein interactions and post-translational modifications, researchers can gain a deeper understanding of the molecular mechanisms underlying diseases. Structural bioinformatics can aid in the interpretation of proteomic data by providing structural models that illustrate how proteins interact within a cellular context. This knowledge is essential for identifying novel therapeutic targets and for designing small molecules or biologics that can specifically disrupt these interactions, leading to more effective treatments.
In addition to target identification and interaction studies, structural bioinformatics contributes to metabolomics, particularly in assessing drug efficacy and safety. By integrating structural data with metabolomic profiles, scientists can evaluate how drug candidates influence metabolic pathways. The ability to visualize how a drug binds to its target can inform predictions about downstream metabolic effects, thereby enabling researchers to assess both the therapeutic and toxicological profiles of new drug candidates early in the development process. This holistic approach enhances the likelihood of successful drug development by ensuring that safety considerations are addressed alongside efficacy.
Lastly, the emergence of machine learning models in drug design is significantly bolstered by structural bioinformatics. By utilizing structural data, machine learning algorithms can be trained to predict the effectiveness of new drug molecules based on their structural features and biological activity. This synergy accelerates the drug discovery process by allowing for rapid evaluation and optimization of compound libraries through virtual screening techniques. By identifying the most promising candidates for further development, researchers can streamline the transition from in silico predictions to experimental validation, ultimately improving the efficiency and success rates of drug development initiatives.
Techniques for Lead Compound Refinement
The refinement of lead compounds is a critical step in the drug discovery process, as it directly influences the efficacy, safety, and specificity of potential therapeutic agents. Various techniques have emerged to enhance the optimization of lead compounds, integrating advancements in bioinformatics with traditional methodologies. These techniques encompass computational approaches, experimental validations, and the incorporation of omics data, all aimed at fine-tuning lead compounds for targeted therapies. By leveraging genomic, proteomic, and metabolomic data, researchers can inform their refinements, leading to improved drug candidates.
One prominent approach in lead compound refinement is the utilization of structural bioinformatics. This field enables scientists to analyze the three-dimensional structures of target proteins and their interactions with lead compounds. Through techniques such as molecular docking and dynamics simulations, researchers can predict binding affinities and optimize the chemical structures of lead candidates. By systematically modifying molecular features and assessing their impact on binding, it is possible to enhance specificity and reduce off-target effects, ultimately leading to safer and more effective drugs.
Machine learning models have also become invaluable in the lead compound optimization process. By analyzing large datasets of biological and chemical information, these algorithms can identify patterns and predict the performance of lead compounds. This predictive modeling not only accelerates the identification of promising candidates but also informs the refinement process by highlighting which molecular characteristics are most critical for effective binding and activity. Furthermore, machine learning can aid in the design of novel compounds that may not have been previously considered, expanding the scope of potential therapeutic options.
Incorporating omics data, such as genomics and proteomics, further enriches the refinement process. Genomic data can reveal potential drug targets by identifying mutations or dysregulations associated with diseases, guiding the selection of lead compounds that are more likely to be effective. Similarly, proteomic analyses can elucidate protein interactions and pathways relevant to drug action, providing insights into how lead compounds can be modified to enhance their therapeutic effects. Metabolomic profiling complements these approaches by assessing the biological impact of drug candidates at the metabolic level, helping to evaluate their efficacy and safety before progressing to clinical trials.
Lastly, the integration of virtual screening techniques allows for the rapid evaluation of extensive compound libraries, significantly reducing the time and resources required for lead compound identification. By using computational methods to predict the interactions between compounds and biological targets, researchers can efficiently narrow down potential candidates for further refinement. This comprehensive approach, combining structural bioinformatics, machine learning, and omics data analysis, ultimately streamlines the drug discovery process, paving the way for more targeted and personalized therapies.
Evaluating Binding Affinity and Specificity
Evaluating binding affinity and specificity is a crucial step in the drug discovery process, particularly in the context of structural bioinformatics. Binding affinity refers to the strength of the interaction between a drug candidate and its target protein, while specificity indicates how selectively a compound interacts with that target compared to others. Understanding these parameters can significantly influence the optimization of lead compounds, as higher binding affinity often correlates with increased therapeutic efficacy, and greater specificity can minimize off-target effects, enhancing the safety profile of new drugs.
To evaluate binding affinity, various experimental techniques such as surface plasmon resonance, isothermal titration calorimetry, and fluorescence polarization can be employed. These methods allow scientists to quantify the interactions between ligands and their targets under different conditions. In parallel, computational approaches are becoming increasingly sophisticated, with molecular docking and dynamics simulations providing insights into the energetics of binding and the conformational changes that may occur upon ligand binding. By integrating these experimental and computational techniques, researchers can refine their understanding of how modifications to lead compounds influence binding affinity.
Specificity assessment often involves evaluating the interactions of a compound with multiple targets. High-throughput screening methods and bioinformatics tools can facilitate this process by allowing for the rapid evaluation of compound libraries against a wide range of biological targets. Advanced proteomic techniques can also be employed to profile off-target interactions, helping to identify potential side effects early in the drug development process. By applying systems biology approaches, scientists can further analyze the complex networks of protein interactions to predict how a drug candidate may behave within the entire biological system, thus enhancing specificity.
Machine learning is emerging as a powerful ally in the evaluation of binding affinity and specificity. By training algorithms on large datasets that include both successful and unsuccessful drug interactions, researchers can predict the likelihood of a compound’s affinity and specificity based on its structural features. These predictive models not only accelerate the screening process but also provide valuable insights into the structural modifications that might improve a compound’s performance. This integration of machine learning with structural bioinformatics represents a paradigm shift in how scientists approach lead compound optimization.
Ultimately, the evaluation of binding affinity and specificity is a multifaceted endeavor that combines experimental techniques, computational modeling, and advanced analytical methods. As the field of bioinformatics continues to evolve, the integration of genomic, proteomic, and metabolomic data will enhance our understanding of drug interactions at a systems level. This holistic approach will not only streamline the drug discovery process but also pave the way for more personalized and effective therapies, addressing the complex challenges of modern pharmacology.
Success Stories in Lead Optimization
Success stories in lead optimization illustrate the transformative power of integrating diverse scientific disciplines in drug discovery. Through advancements in bioinformatics, researchers have successfully harnessed genomic data analysis, proteomics, and metabolomics, among other technologies, to identify and refine potential drug candidates. These stories not only highlight the efficacy of interdisciplinary approaches but also underscore the importance of collaboration in overcoming the complexities inherent in drug development.
One notable success story involves the use of genomic data in identifying drug targets for complex diseases such as cancer. By analyzing large-scale genomic datasets, researchers identified mutations that drive tumorigenesis, leading to the development of targeted therapies. The integration of bioinformatics tools allowed for the identification of novel biomarkers, facilitating the design of lead compounds that specifically inhibit these mutations. This targeted approach not only enhanced the selectivity of the drugs but also significantly improved patient outcomes, illustrating the power of genomic data in rational drug design.
Proteomics has also played a crucial role in optimizing lead compounds. By employing advanced proteomic techniques, researchers have gained insights into protein interactions and their implications for drug action. For instance, a study focused on a specific cancer type revealed a network of interacting proteins that were critical for tumor survival. By utilizing this information, scientists were able to design lead compounds that disrupted these interactions, leading to effective therapeutic agents. The ability to map protein networks and understand their dynamics has proven invaluable in refining lead compounds to achieve desired biological effects.
Metabolomics offers another layer of insight into drug efficacy assessment. By profiling the metabolic changes induced by drug candidates, researchers can evaluate not only the therapeutic effects but also the safety profiles of these compounds. A successful application of this approach was seen in the development of a novel analgesic. Metabolomic analysis revealed specific metabolic pathways that were modulated by the drug, allowing scientists to fine-tune the compound for optimal efficacy while minimizing adverse effects. This holistic understanding of drug action through metabolomic profiling is becoming increasingly recognized as a critical step in lead optimization.
Lastly, the incorporation of machine learning models in drug design has revolutionized how lead compounds are identified and optimized. By analyzing vast datasets of biological information, machine learning algorithms can predict the effectiveness of new drug molecules with remarkable accuracy. A recent project demonstrated this by leveraging machine learning to sift through a library of compounds, identifying candidates with the highest potential for binding affinity to a target protein. This not only accelerated the drug discovery process but also led to the identification of previously overlooked compounds, showcasing the potential of computational methods in enhancing lead optimization efforts.
In conclusion, these success stories underscore the impact of integrating various scientific approaches in lead optimization. By leveraging genomic data, proteomics, metabolomics, and machine learning, researchers are better equipped to navigate the complexities of drug discovery. The collaborative nature of these efforts not only enhances the likelihood of developing effective therapies but also paves the way for personalized medicine, ultimately improving patient care and outcomes. As the field of structural bioinformatics continues to evolve, the lessons learned from these successes will undoubtedly inform future strategies in optimizing lead compounds for targeted therapies.
Chapter 7: Systems Biology Approaches in Pharmacology
Introduction to Systems Biology
Systems biology is an interdisciplinary field that merges biology, computer science, and engineering to understand the complex interactions within biological systems. This subchapter serves as an introduction to systems biology, emphasizing its significance in drug discovery and development. By leveraging quantitative methods and computational modeling, systems biology allows researchers to analyze biological data holistically, leading to a more comprehensive understanding of cellular processes and disease mechanisms. This approach is particularly vital in addressing the multifaceted nature of diseases, where multiple biological pathways and interactions are often involved.
At the core of systems biology is the integration of diverse data types, including genomic, proteomic, and metabolomic information. This amalgamation enables scientists to identify potential drug targets with greater accuracy. For instance, genomic data analysis plays a crucial role in pinpointing genetic mutations that drive diseases, thus providing a basis for targeted therapies. When combined with proteomic techniques, which elucidate protein interactions and their functional roles, researchers can gain insights into the molecular underpinnings of diseases, allowing for the development of more effective therapeutic strategies.
Moreover, systems biology enhances the evaluation of drug efficacy through metabolomic profiling. By assessing the metabolic changes that occur in response to drug treatment, scientists can determine the safety and effectiveness of new drug candidates. This information is essential for refining lead compounds, ensuring that they not only interact favorably with their targets but also elicit the desired biological responses without adverse effects. The integration of metabolomics into drug discovery underscores the importance of understanding how drugs alter biological pathways at the metabolic level.
The advent of machine learning has further amplified the potential of systems biology in drug design. By developing algorithms that can analyze large datasets, researchers are now able to predict the effectiveness of new drug molecules based on their biological profiles. These predictive models can streamline the drug discovery process by identifying promising candidates early in development, thus reducing time and costs associated with traditional screening methods. As a result, the application of machine learning within the frameworks of systems biology holds the promise of revolutionizing how new drugs are designed and optimized.
In summary, systems biology offers a robust framework for addressing the complexities of drug discovery and development. Its integrative approach allows scientists to navigate the intricate biological networks involved in diseases, ultimately enhancing the identification of drug targets, refining lead compounds, and evaluating drug efficacy. As the field progresses, the continued collaboration between systems biology and bioinformatics will pave the way for more personalized and effective therapeutic interventions, aligning with the future of medicine that prioritizes individualized patient care.
Integrating Systems Biology in Drug Discovery
Integrating systems biology in drug discovery represents a paradigm shift in how scientists approach the identification and optimization of new therapeutic compounds. This interdisciplinary field combines biological data with computational models to understand the complex interactions within biological systems. By leveraging systems biology, researchers can develop a holistic understanding of disease mechanisms, which is crucial for discovering novel drug targets and improving the efficacy of existing therapies. This approach emphasizes the importance of context in biological research, making it possible to identify not just potential drug targets but also the pathways and networks that influence their behavior.
Genomic data analysis plays a pivotal role in systems biology, particularly in identifying drug targets for various diseases. By integrating genomic information from patient samples, researchers can identify gene mutations and expression patterns that drive disease progression. This data-driven approach allows for the identification of specific molecular targets that can be exploited for therapeutic intervention. In addition, the incorporation of genomic analysis with other omics technologies, such as proteomics and metabolomics, provides a more comprehensive view of the biological landscape, thus enhancing the drug discovery process.
Proteomics, the large-scale study of proteins and their functions, complements genomic data by providing insights into protein interactions and post-translational modifications. Understanding these interactions is crucial for drug development, as the efficacy of a drug often depends on its ability to bind to specific proteins within a biological network. Systems biology approaches enable researchers to map these intricate protein-protein interactions and identify potential off-target effects, thereby optimizing lead compounds for better therapeutic outcomes. This integration of proteomic data into systems biology frameworks is essential for developing drugs that are both effective and safe.
Metabolomics adds another layer of complexity to drug discovery by allowing scientists to evaluate the effects of drug candidates on metabolic pathways. By profiling metabolites in biological samples, researchers can assess the pharmacodynamics and pharmacokinetics of new drugs. Systems biology frameworks facilitate the interpretation of metabolomic data in the context of genetic and proteomic information, enabling a more accurate assessment of a drug's efficacy and safety. This holistic evaluation can lead to the early identification of potential adverse effects, thereby improving the overall drug development process.
The application of machine learning models within systems biology further enhances drug discovery efforts. By analyzing large datasets derived from genomic, proteomic, and metabolomic studies, machine learning algorithms can predict the effectiveness of new drug molecules based on biological data. These predictive models can identify promising lead compounds and streamline the optimization process, ultimately accelerating the transition from bench to bedside. As researchers continue to integrate systems biology with advanced computational techniques, the potential for discovering innovative therapies tailored to individual patient profiles becomes increasingly feasible, revolutionizing the landscape of drug discovery and personalized medicine.
Complex Interactions and Their Implications
Complex interactions among biological molecules are central to understanding the intricacies of drug discovery and development. These interactions often involve multiple layers of molecular communication, where proteins, nucleic acids, and metabolites engage in a dynamic network that influences cellular functions. In drug discovery, recognizing these complex interactions is crucial for identifying potential drug targets and assessing the efficacy of new compounds. By employing advanced techniques in bioinformatics, scientists can unravel these molecular interdependencies and translate their findings into optimized therapeutic strategies.
Genomic data analysis plays a pivotal role in identifying drug targets by providing insights into genetic variations that contribute to disease pathways. High-throughput sequencing technologies generate vast amounts of genomic information, which can be harnessed to pinpoint mutations or expression changes in specific genes associated with various diseases. By integrating genomic data with proteomic and metabolomic analyses, researchers can create a comprehensive map of the molecular landscape underlying a disease, enabling the identification of novel targets for therapeutic intervention. This holistic approach not only enhances the understanding of disease mechanisms but also informs the design of targeted therapies that can improve patient outcomes.
Proteomics adds another dimension to the study of complex interactions by focusing on the protein level, where the majority of drug action occurs. Understanding protein-protein interactions, post-translational modifications, and the dynamic behavior of protein complexes is essential for drug development. Advanced proteomic techniques, such as mass spectrometry and affinity purification, allow for the detailed characterization of these interactions, revealing potential pathways that can be exploited for therapeutic purposes. By elucidating the roles of specific proteins in disease processes, scientists can better design lead compounds that selectively target these proteins, enhancing drug specificity and minimizing off-target effects.
Metabolomics serves as a powerful tool for evaluating drug efficacy and safety by profiling small-molecule metabolites within biological systems. This discipline provides insights into the biochemical changes that occur in response to drug treatment, offering a real-time view of how compounds affect cellular metabolism. By analyzing metabolomic data, researchers can identify biomarkers of drug response, which can be critical in assessing the therapeutic potential of new drug candidates. Furthermore, metabolomic profiling can uncover unintended metabolic consequences of drug administration, guiding the refinement of lead compounds for improved safety profiles.
Machine learning models have emerged as essential tools in the optimization of lead compounds, facilitating the prediction of drug efficacy based on biological data. By training algorithms on vast datasets derived from genomic, proteomic, and metabolomic analyses, researchers can uncover patterns that inform the design and selection of novel drug candidates. These models can also integrate data from various sources, including clinical trials, to enhance the predictive power of drug design processes. As machine learning continues to evolve, its application in structural bioinformatics will become increasingly vital for optimizing lead compounds, ultimately accelerating the drug discovery timeline and improving therapeutic outcomes in personalized medicine.
Case Studies: Systems Biology in Action
Case studies in the realm of systems biology provide valuable insights into the practical applications of bioinformatics technologies in drug discovery and development. By examining specific examples, researchers can illuminate how genomic data analysis, proteomics, metabolomics, and machine learning are effectively utilized to optimize lead compounds and identify new therapeutic targets. These case studies serve as a testament to the integration of various bioinformatics tools and methodologies, demonstrating their collective power in advancing pharmacological research.
One prominent case study involves the application of genomic data analysis in identifying drug targets for cancer therapies. Researchers utilized large-scale genomic sequencing data to uncover mutations and dysregulated pathways in tumor samples. By integrating this genomic information with bioinformatics tools, they identified several novel targets that were previously overlooked. This systematic integration not only accelerated the drug discovery process but also provided a more precise understanding of the tumor microenvironment, ultimately leading to the development of targeted therapies that exhibited enhanced efficacy against specific cancer types.
In the field of proteomics, another compelling case study highlights the use of proteomic techniques to elucidate protein-protein interactions that are critical in disease progression. Through quantitative mass spectrometry, scientists were able to profile the proteomes of diseased versus healthy tissues, identifying key differences in protein expression and interaction networks. This approach revealed potential biomarkers for disease and novel therapeutic targets, guiding the design of new drugs aimed at modulating these interactions. The insights gained from this proteomic analysis significantly contributed to the development of biologics that selectively target disease-associated proteins.
Metabolomics has also emerged as a crucial component in assessing drug efficacy. A notable case study showcased how metabolomic profiling was employed to evaluate the safety and effectiveness of a new anti-inflammatory drug. By analyzing the metabolic changes in patients undergoing treatment, researchers identified specific metabolites that correlated with therapeutic outcomes. This data not only helped in understanding the drug's mechanism of action but also provided a framework for predicting patient responses. Such metabolomic approaches facilitate the identification of biomarkers for drug efficacy, ultimately informing clinical decision-making and personalized medicine strategies.
The integration of machine learning models into drug design has transformed the landscape of pharmacology. A case study illustrates the development of a machine learning algorithm that predicted the binding affinities of novel drug candidates based on extensive biological data. By training the model on existing datasets of known compounds and their interactions, researchers achieved a high degree of accuracy in forecasting the effectiveness of new molecules. This predictive capability not only streamlines the drug discovery process but also enhances the potential for identifying lead compounds that may have otherwise been overlooked in traditional screening methods.
These case studies exemplify the multifaceted applications of systems biology in pharmacology, showcasing how bioinformatics technologies are revolutionizing drug discovery and development. By leveraging genomic, proteomic, and metabolomic data along with advanced computational techniques, researchers are equipped to tackle the complexities of biological systems, leading to more effective and targeted therapeutic strategies. As the field continues to evolve, the integration of these diverse approaches will undoubtedly yield further innovations in optimizing lead compounds and improving patient outcomes.
Chapter 8: Virtual Screening Techniques for Compound Library Exploration
Overview of Virtual Screening
Virtual screening is an essential computational technique in drug discovery that enables scientists to evaluate vast libraries of compounds quickly and efficiently. By harnessing the power of computational biology, researchers can predict how small molecules interact with biological targets, such as proteins implicated in various diseases. This approach not only accelerates the identification of potential drug candidates but also reduces the costs associated with experimental screening methods. The integration of virtual screening into the drug discovery pipeline has proven particularly beneficial in the early stages of lead identification and optimization.
The methodology behind virtual screening typically involves two main types: structure-based and ligand-based approaches. Structure-based virtual screening utilizes the three-dimensional structures of biological targets, derived from techniques such as X-ray crystallography or nuclear magnetic resonance (NMR) spectroscopy, to evaluate how well potential drug molecules fit into the binding sites of these targets. On the other hand, ligand-based virtual screening relies on existing knowledge of known active compounds to identify new candidates with similar properties. Both approaches leverage sophisticated algorithms and scoring functions to assess binding affinities and predict the likelihood of success in subsequent biological assays.
One of the key advantages of virtual screening is its ability to facilitate the exploration of compound libraries that would be impractical to screen experimentally. With the advent of high-throughput screening technologies, researchers can generate extensive datasets of chemical compounds. Virtual screening allows for the prioritization of these compounds based on predicted biological activity, thereby streamlining the drug discovery process. This is especially relevant in the context of genomic data analysis, where the identification of drug targets can be enhanced by virtual screening techniques that align potential compounds with specific genetic profiles associated with diseases.
Moreover, the application of machine learning models in virtual screening is transforming the landscape of drug design. By training algorithms on large datasets that encompass various biological and chemical properties, scientists can create predictive models that assess the likelihood of a compound's effectiveness. This intersection of virtual screening and machine learning not only increases the accuracy of predictions but also aids in the identification of novel drug candidates that may have been overlooked through traditional methods. In this way, virtual screening acts as a bridge between bioinformatics and experimental pharmacology, fostering a more integrated approach to drug discovery.
In conclusion, the role of virtual screening in structural bioinformatics is integral to optimizing lead compounds for targeted therapies. By efficiently narrowing down potential drug candidates from extensive libraries, virtual screening complements genomic data analysis, proteomics, metabolomics, and systems biology approaches. As the field continues to evolve, the incorporation of advanced computational techniques and machine learning will further enhance the effectiveness of virtual screening, ultimately leading to more successful drug discovery outcomes and improved therapeutic strategies.
Methods and Approaches in Virtual Screening
Virtual screening is a pivotal aspect of drug discovery that leverages computational techniques to evaluate vast libraries of compounds for potential therapeutic efficacy. This method allows scientists to identify promising drug candidates without the need for extensive laboratory work, thus accelerating the initial phases of drug development. By employing various computational algorithms and molecular modeling tools, virtual screening can predict the interactions between small molecules and biological targets, typically proteins implicated in diseases. The growing integration of bioinformatics technologies in virtual screening has revolutionized traditional drug discovery approaches, making them more efficient and cost-effective.
One of the primary methods used in virtual screening is ligand-based screening, which relies on the known interactions of existing compounds to identify similar candidates within large databases. Techniques such as quantitative structure-activity relationship (QSAR) modeling play a crucial role in this context, allowing researchers to correlate chemical structure with biological activity. By analyzing the features of known active compounds, scientists can generate predictive models that facilitate the identification of new lead compounds. This method is particularly beneficial when the three-dimensional structure of the target protein is not available, as it focuses on the chemical properties of potential ligands rather than their interactions with a specific target.
Another significant approach is structure-based virtual screening, which involves the use of the three-dimensional structures of biological targets to identify potential inhibitors. Molecular docking is a key technique in this method, where compounds are computationally "docked" into the binding site of a target protein to assess their binding affinity and orientation. This information helps in predicting which compounds are likely to exhibit the desired biological activity. The advancements in cryo-electron microscopy and X-ray crystallography have made high-resolution structures of various proteins accessible, further enhancing the accuracy of structure-based screening methods.
Machine learning models have emerged as a transformative force in virtual screening, enabling the analysis of complex biological data to predict drug efficacy. These algorithms can learn from vast datasets, identifying patterns and correlations that may not be apparent through traditional analysis methods. By integrating genomic, proteomic, and metabolomic data, machine learning can facilitate a more holistic understanding of drug interactions and their biological implications. This approach not only improves the accuracy of virtual screening but also allows for the development of predictive models that can guide the optimization of lead compounds.
In summary, the methods and approaches in virtual screening are essential for streamlining the drug discovery process. By combining ligand-based and structure-based techniques with the power of machine learning and bioinformatics, researchers can efficiently explore compound libraries and identify potential drug candidates. As the field continues to evolve, the integration of multidisciplinary approaches, including genomic data analysis, proteomics, and systems biology, will further enhance the capabilities of virtual screening, ultimately leading to the development of more effective and targeted therapies.
Evaluating Compound Libraries Efficiently
Evaluating compound libraries efficiently is a critical step in the drug discovery process, particularly within the realm of structural bioinformatics. The sheer volume of potential drug candidates present in compound libraries necessitates the use of systematic approaches to streamline evaluation and select compounds with the highest likelihood of success in therapeutic applications. By integrating bioinformatics tools and methodologies, researchers can enhance the accuracy and speed of their evaluations, ultimately leading to more effective targeted therapies.
One of the primary strategies employed in the efficient evaluation of compound libraries is virtual screening. This approach utilizes computational methods to predict the interactions between compounds and their biological targets, allowing scientists to assess vast libraries without the need for exhaustive wet-lab experiments. Techniques such as molecular docking and molecular dynamics simulations can provide insights into binding affinities and specificities, helping to prioritize compounds that are most likely to exhibit desired biological activity. As these tools continue to advance, they play an increasingly pivotal role in the drug discovery pipeline.
In addition to virtual screening, the integration of genomic data analysis offers a powerful means of identifying potential drug targets. By leveraging large-scale genomic datasets, researchers can gain insights into the genetic basis of diseases and pinpoint the molecular targets that are most amenable to pharmacological intervention. This targeted approach not only enhances the efficiency of compound library evaluation but also ensures that the compounds selected are relevant to the specific disease pathology, thereby increasing the likelihood of clinical success.
Proteomics and metabolomics further enrich the compound evaluation process by providing a comprehensive understanding of protein interactions and metabolic responses to drug candidates. Proteomic techniques can elucidate the interactions between proteins and compounds, revealing potential off-target effects or synergistic interactions that may influence drug efficacy. Similarly, metabolomic profiling can assess the biological impact of compounds at the cellular level, offering insights into their safety and effectiveness. By incorporating these approaches, scientists can make more informed decisions about which compounds to advance for further testing.
Machine learning models have emerged as invaluable tools in the evaluation of compound libraries, enabling researchers to predict the effectiveness of new drug molecules based on vast biological datasets. By training algorithms on existing data, machine learning can uncover patterns and relationships that may not be immediately apparent through traditional analysis. This predictive capability allows for the prioritization of compounds with the highest probability of success, thus optimizing lead compound selection. Furthermore, the integration of systems biology approaches facilitates a holistic understanding of the complex interactions within biological systems, enhancing the predictive power of these models and leading to more successful drug development outcomes.
Real-World Applications of Virtual Screening
Real-world applications of virtual screening have revolutionized the landscape of drug discovery and development. By employing computational techniques to assess vast libraries of compounds, researchers can efficiently identify potential drug candidates that target specific biological pathways. This approach not only accelerates the initial stages of drug discovery but also enhances the precision with which researchers can select compounds for further testing. As the demand for novel therapeutics continues to rise, virtual screening stands out as a critical tool in the biopharmaceutical arsenal, enabling scientists to streamline their efforts in developing effective treatments for various diseases.
One prominent application of virtual screening lies in genomic data analysis for drug target identification. Through the integration of genomic information, researchers can pinpoint gene mutations and expression patterns associated with specific diseases. Virtual screening methodologies can then be applied to identify compounds that interact with these identified targets. This synergy between genomic data and virtual screening not only narrows down the search for drug candidates but also improves the likelihood of success by focusing on biologically relevant interactions. Such targeted approaches are particularly valuable in addressing complex diseases like cancer, where multiple pathways often contribute to disease progression.
Proteomics also plays a significant role in the application of virtual screening. By utilizing proteomic techniques, scientists can explore protein interactions, post-translational modifications, and signaling pathways that are crucial for drug action. Virtual screening can be employed to model these protein-ligand interactions, allowing researchers to predict how new compounds might affect biological systems. This integration of proteomics and virtual screening enhances the understanding of how potential drugs might work at the molecular level, providing insights that are essential for optimizing lead compounds and improving their therapeutic potential.
Metabolomics further enriches the application of virtual screening by providing a comprehensive view of metabolic changes induced by drug candidates. By profiling metabolites in response to drug treatment, researchers can assess the efficacy and safety of new compounds in a biological context. Virtual screening techniques can facilitate the identification of compounds that not only bind effectively to their targets but also induce favorable metabolic responses. This holistic approach to evaluating drug candidates ensures that potential therapies are not only effective but also safe, paving the way for more informed decision-making in drug development.
Finally, machine learning models significantly enhance virtual screening by enabling the prediction of drug effectiveness based on large datasets. As machine learning algorithms become increasingly sophisticated, they can analyze complex biological data to identify patterns that inform drug design. This allows for the development of more targeted virtual screening strategies that can predict the likelihood of success for various compounds. When combined with systems biology approaches, these models provide a comprehensive framework for understanding the intricate interactions within biological systems, further optimizing the drug discovery process. Collectively, the real-world applications of virtual screening reflect its vital role in advancing the science of drug discovery, ultimately leading to more effective and personalized therapeutic options.
Chapter 9: Bioinformatics Tools for Drug Repurposing
Introduction to Drug Repurposing
Drug repurposing, also known as drug repositioning, is a strategic approach in pharmacology that involves identifying new therapeutic uses for existing drugs. This concept has gained significant traction in recent years, particularly due to the high costs and lengthy timelines associated with traditional drug discovery processes. By leveraging drugs that have already undergone extensive testing for safety and efficacy, researchers can potentially accelerate the availability of new treatments for various diseases. The integration of bioinformatics technologies plays a pivotal role in this endeavor, enabling scientists to analyze vast datasets and uncover novel therapeutic opportunities.
The application of genomic data analysis is essential for identifying potential drug targets in the context of drug repurposing. By examining genetic variations and expression levels associated with specific diseases, researchers can pinpoint existing drugs that may interact with these targets. This approach not only streamlines the drug development process but also enhances the likelihood of successful outcomes, as repurposed drugs are often better understood in terms of their pharmacodynamics and pharmacokinetics. Furthermore, genomic insights can guide the selection of patient populations most likely to benefit from these therapies, thus facilitating personalized medicine initiatives.
Proteomics also plays a critical role in drug repurposing by providing insights into protein interactions and signaling pathways that may be altered in disease states. High-throughput proteomic techniques allow for the identification of biomarkers and therapeutic targets, which can be invaluable when searching for existing drugs that might exhibit unexpected efficacy. By understanding the protein landscape associated with various diseases, researchers can more effectively evaluate the potential of repurposed drugs to modulate these pathways and restore homeostasis.
Metabolomics offers additional layers of information that can aid in assessing drug efficacy and safety during the repurposing process. By analyzing metabolic profiles, researchers can gauge the biological impact of existing drugs on cellular metabolism and identify potential off-target effects. This comprehensive understanding of how drugs influence metabolic networks can inform decisions about which candidates are most promising for further development. Integrating metabolomic data with other omics approaches enhances the overall robustness of drug repurposing efforts.
Machine learning models have emerged as powerful tools in the drug repurposing landscape, enabling researchers to predict the effectiveness of existing drugs based on biological data. By training algorithms on large datasets that encompass genomic, proteomic, and metabolomic information, scientists can identify patterns that might not be apparent through traditional analysis. These predictive models can streamline the identification of repurposed drug candidates, thereby optimizing the drug discovery pipeline. As the field of structural bioinformatics continues to evolve, the integration of these advanced computational techniques will be instrumental in driving the next generation of drug repurposing strategies.
Bioinformatics Approaches for Identifying Repurposing Opportunities
Bioinformatics approaches have emerged as pivotal tools in the identification of drug repurposing opportunities, leveraging vast amounts of biological data to uncover new therapeutic applications for existing drugs. The integration of genomic, proteomic, and metabolomic data allows researchers to explore complex biological systems more effectively. By harnessing these diverse datasets, scientists can elucidate the mechanisms of action of known compounds, facilitating the discovery of novel uses for medications that have already undergone safety evaluations. This not only accelerates the drug development process but also provides a cost-effective strategy to address unmet medical needs, particularly in the context of emerging diseases.
Genomic data analysis plays a crucial role in drug target identification, offering insights into the genetic underpinnings of diseases. By analyzing variations in gene expression, mutations, and other genomic features, bioinformatics tools can highlight potential targets for drug action. For example, researchers can utilize bioinformatics algorithms to identify genes that are differentially expressed in disease states compared to healthy controls. Once potential drug targets are identified, existing compounds can be mapped against these targets, revealing opportunities for repurposing. This genomic-focused approach not only enhances the understanding of disease biology but also streamlines the identification of new therapeutic uses for existing drugs.
Proteomics further enriches the drug repurposing landscape by providing detailed information about protein interactions and pathways involved in disease processes. Advanced proteomic technologies, such as mass spectrometry, enable the characterization of protein expression profiles and post-translational modifications. By integrating proteomic data with genomic findings, researchers can construct comprehensive interaction networks that reveal how existing drugs may influence various signaling pathways. This systems-level understanding is critical for identifying repurposing opportunities, as it allows for the prediction of off-target effects and potential synergies with other therapeutic agents.
In addition to genomic and proteomic data, metabolomics plays a vital role in assessing the efficacy and safety of repurposed drugs. Metabolomic profiling involves the comprehensive analysis of metabolites within biological systems, providing insights into biochemical changes associated with drug treatment. By comparing metabolomic profiles before and after drug administration, researchers can evaluate the impact of existing drugs on metabolic pathways relevant to specific diseases. This information is invaluable for identifying drugs that not only show promise in novel indications but also maintain favorable safety profiles, thus enhancing the likelihood of successful repurposing.
Machine learning models represent the cutting edge of bioinformatics in drug repurposing, allowing for the rapid analysis of complex biological data. These algorithms can predict the effectiveness of existing drug molecules based on patterns within genomic, proteomic, and metabolomic data. By training models on known drug-target interactions and their therapeutic outcomes, researchers can generate hypotheses for potential repurposing candidates. This data-driven approach complements traditional experimental methods, enabling scientists to prioritize compounds for further investigation and ultimately accelerating the journey from bench to bedside. The convergence of bioinformatics technologies and machine learning thus holds significant promise for transforming drug discovery and development through efficient repurposing strategies.
Case Studies: Successful Drug Repurposing
Case studies of successful drug repurposing provide valuable insights into the intersection of bioinformatics and pharmacology, revealing how existing drugs can be repositioned to treat new diseases. One prominent example is the repurposing of the antiviral drug Sofosbuvir, originally developed to treat hepatitis C, which has shown efficacy against other viral infections, including COVID-19. By employing genomic data analysis, researchers were able to identify common pathways and mechanisms shared by these viruses, leading to the strategic use of Sofosbuvir in treating patients affected by COVID-19. This case exemplifies the power of integrating genomic data in drug target identification, allowing for rapid adaptation of therapies to emerging health crises.
Another significant case is the repurposing of thalidomide, a drug notorious for its teratogenic effects, which has found new life in treating multiple myeloma and leprosy. Advances in proteomics enabled scientists to delve deeper into the protein interactions influenced by thalidomide, uncovering its effects on the immune system and angiogenesis. By characterizing these interactions, researchers could devise protocols that mitigate the drug’s adverse effects while maximizing its therapeutic benefits. This case highlights the importance of proteomic techniques in understanding the multifaceted roles of existing drugs and their implications in drug development.
In the realm of oncology, the use of metformin, a common diabetes medication, has garnered attention for its potential anti-cancer properties. Metabolomic profiling has played a crucial role in assessing its efficacy and safety across various cancer types. By analyzing metabolic changes in cancer cells treated with metformin, researchers were able to elucidate the drug's impact on cellular metabolism and its potential to improve outcomes when combined with other therapies. This case study underscores how metabolomics can inform drug efficacy assessment, providing a robust framework for evaluating existing drugs in new therapeutic contexts.
Machine learning models have also emerged as powerful tools in the drug repurposing landscape. An illustrative example is the use of machine learning algorithms to predict the effectiveness of existing compounds for treating Alzheimer's disease. By training models on vast biological datasets, researchers identified novel drug candidates that may have been overlooked in traditional screening methods. This case emphasizes the transformative role of machine learning in drug design, allowing for the rapid identification and validation of repurposed drugs with high potential for success.
Finally, the integration of systems biology approaches has proven instrumental in understanding the complex interactions within biological systems during drug discovery. One case that stands out is the repurposing of existing anti-inflammatory drugs to treat chronic pain conditions. By employing a systems biology framework, researchers mapped the intricate signaling networks involved in pain modulation, identifying existing drugs that could target multiple nodes within these networks. This comprehensive approach not only enhances the understanding of drug mechanisms but also paves the way for more effective therapies in personalized medicine. Collectively, these case studies illustrate the significant advancements made through bioinformatics in the realm of drug repurposing, opening new avenues for therapeutic innovation.
Future Directions in Drug Repurposing
Future directions in drug repurposing are poised to significantly transform the landscape of drug development, leveraging advances in bioinformatics and related technologies. As the need for rapid therapeutic solutions continues to grow, particularly in the face of emerging diseases and antibiotic resistance, drug repurposing offers a pragmatic approach to expedite the availability of effective treatments. This subchapter explores innovative strategies and technologies that promise to enhance the repurposing of existing drugs for new therapeutic indications.
One of the most promising avenues for drug repurposing lies in the integration of genomic data analysis with bioinformatics tools. By harnessing vast datasets derived from genomic sequencing, researchers can identify novel drug targets associated with various diseases. This approach not only streamlines the target identification process but also enables the discovery of unexpected therapeutic uses for existing compounds. The ability to correlate genetic variations in patients with drug response offers a robust framework for identifying candidates that may be suitable for repurposing, ultimately accelerating the transition from bench to bedside.
Proteomics is another critical component of future drug repurposing strategies. Advanced proteomic techniques allow for the comprehensive examination of protein interactions and post-translational modifications, which are essential in understanding the molecular mechanisms underlying disease processes. By employing proteomic profiling, researchers can uncover biomarkers of drug efficacy and safety, thereby informing the selection of existing drugs that could be repurposed based on their interaction profiles. This deep understanding of protein dynamics is crucial for predicting how existing drugs will behave in new therapeutic contexts.
The application of machine learning models in drug design is rapidly evolving, creating new opportunities for drug repurposing. Machine learning algorithms can analyze complex biological data to predict the effectiveness of drug molecules against specific targets with greater accuracy. By training models on diverse datasets that include genomic, proteomic, and clinical trial data, researchers can identify potential repurposing candidates that may have been overlooked in traditional screening processes. These predictive capabilities not only enhance the efficiency of drug discovery but also facilitate the systematic exploration of large compound libraries for repurposing opportunities.
Finally, the integration of systems biology approaches into pharmacology is essential for understanding the multifaceted interactions within biological systems during drug repurposing efforts. Systems biology provides a holistic view of how drugs interact with various components of cellular networks, allowing researchers to evaluate the broader implications of repurposing existing drugs. By employing virtual screening techniques alongside systems biology models, scientists can rapidly assess the potential of existing compounds, ensuring that the most promising candidates are prioritized for further development. Together, these advancements in bioinformatics and related fields signify a transformative shift in how we approach drug discovery and repurposing in the future, ultimately leading to more efficient and targeted therapies.
Chapter 10: Personalized Medicine and Bioinformatics
The Concept of Personalized Medicine
The concept of personalized medicine represents a transformative approach in healthcare, particularly in the realm of drug discovery and development. This paradigm shift is predicated on the understanding that individual genetic, environmental, and lifestyle factors influence patient responses to therapies. In the context of bioinformatics, this means harnessing vast amounts of genomic, proteomic, and clinical data to tailor treatments that are not only effective but also minimize adverse effects. Personalized medicine seeks to move away from the traditional one-size-fits-all model, allowing for more precise interventions that align with the unique biological makeup of each patient.
Genomic data analysis plays a pivotal role in identifying potential drug targets for various diseases. By sequencing and analyzing the genomes of individuals, researchers can pinpoint genetic variations that contribute to disease susceptibility and drug response. This information can lead to the identification of novel biomarkers that serve as targets for drug development. For instance, mutations in specific genes can indicate how a patient will metabolize a drug, thereby guiding the selection of the most appropriate therapeutic agents. The integration of genomic data into the drug discovery process not only enhances target identification but also supports the justification of clinical trial designs tailored to specific patient populations.
Proteomics, the large-scale study of proteins, further enriches the personalized medicine landscape by elucidating protein interactions and their implications in drug development. Understanding the proteome—the complete set of proteins expressed in a given cell or organism—provides insights into the mechanisms of action of drugs and the pathways they affect. By employing advanced proteomic techniques, scientists can investigate how individual variations in protein expression influence drug efficacy and safety. This knowledge is crucial for developing targeted therapies that consider the patient's unique protein profile, thereby optimizing treatment regimens and enhancing patient outcomes.
Metabolomics complements genomic and proteomic studies by profiling small molecules in biological samples, offering a comprehensive view of metabolic changes associated with disease and drug treatment. This field allows for the assessment of drug efficacy and safety through the evaluation of metabolic responses to therapeutic interventions. By analyzing metabolites, researchers can identify biomarkers that reflect the pharmacological effects of drugs, enabling a more nuanced understanding of how different patients may respond to treatment. Integrating metabolomics into personalized medicine can improve the prediction of drug responses and facilitate the development of more effective therapeutic strategies.
Finally, the application of machine learning models in drug design is revolutionizing personalized medicine by enabling the prediction of drug effectiveness based on biological data. Machine learning algorithms can analyze complex datasets from genomic, proteomic, and metabolomic studies, identifying patterns that inform drug development processes. By integrating these data streams, researchers can develop models that predict how individual patients will respond to specific drug compounds, thereby supporting the design of tailored therapies. This approach not only accelerates the drug discovery pipeline but also enhances the precision of therapeutic interventions, ultimately contributing to the realization of personalized medicine as a cornerstone of modern healthcare.
Role of Bioinformatics in Tailoring Therapies
The role of bioinformatics in tailoring therapies has become increasingly significant in the realm of drug discovery and development. As the complexity of biological systems is unveiled, bioinformatics serves as a crucial tool for analyzing vast amounts of genomic, proteomic, and metabolomic data. By leveraging advanced computational techniques, scientists can identify potential drug targets, optimize lead compounds, and personalize therapies to enhance efficacy and minimize adverse effects. This integration of bioinformatics into the drug development pipeline represents a transformative shift towards more targeted and effective treatments.
One of the primary applications of bioinformatics in tailoring therapies is genomic data analysis, which plays a pivotal role in drug target identification. By examining genomic sequences and variations, researchers can pinpoint genes and pathways implicated in specific diseases. These insights enable the identification of novel drug targets that are more likely to yield effective therapies. Furthermore, bioinformatics tools facilitate the integration of genomic data with clinical outcomes, allowing scientists to better understand the relationship between genetic factors and disease susceptibility, which is essential for developing personalized treatment strategies.
Proteomics also benefits significantly from bioinformatics, as it provides a framework for understanding protein interactions and their implications in drug development. Through the use of bioinformatics algorithms, researchers can analyze large-scale proteomic data to identify biomarkers and elucidate the molecular mechanisms underlying drug action. This understanding is critical for optimizing lead compounds, as it enables scientists to design drugs that specifically target protein interactions involved in disease pathways. By utilizing structural bioinformatics, researchers can refine the binding affinity and specificity of lead compounds, ultimately enhancing their therapeutic potential.
Metabolomics offers another layer of insight into drug efficacy assessment, where bioinformatics tools play a vital role in analyzing metabolic profiles. By comparing the metabolomic signatures of treated and untreated subjects, researchers can evaluate the safety and effectiveness of new drug candidates. This approach not only aids in identifying potential side effects but also provides information on the metabolic pathways affected by the drug, thereby informing dosage and treatment regimens. The integration of metabolomic data into the drug development process ensures a comprehensive assessment of a drug's impact on biological systems.
Machine learning models have emerged as powerful tools in drug design, enabling the prediction of new drug molecules' effectiveness based on biological data. Bioinformatics facilitates the development of these models by providing high-quality datasets that encompass genomic, proteomic, and clinical information. The ability to analyze complex interactions within biological systems through systems biology approaches further enhances the predictive capabilities of machine learning algorithms. As a result, bioinformatics not only streamlines the identification of promising drug candidates but also supports the ongoing refinement of therapies tailored to individual patient profiles, ultimately paving the way for advancements in personalized medicine.
Genetic Profiling and Drug Response
Genetic profiling has emerged as a pivotal element in the realm of drug response, influencing both the discovery and development of targeted therapies. By analyzing an individual's genetic makeup, researchers can identify specific genetic variations that affect how patients metabolize and respond to drugs. This personalized approach not only enhances the efficacy of treatments but also minimizes adverse effects, thereby supporting the overarching goal of personalized medicine. As scientists increasingly integrate genomic data into the drug development pipeline, understanding the genetic basis of drug response becomes critical for optimizing therapeutic strategies.
The identification of drug targets through genomic data analysis is a key aspect of modern pharmacogenomics. By leveraging large-scale genomic datasets, researchers can pinpoint mutations and polymorphisms that contribute to disease pathology and drug resistance. This process involves sophisticated bioinformatics tools that analyze gene expression profiles, single nucleotide polymorphisms (SNPs), and copy number variations. Identifying potential drug targets at the genomic level allows for the development of more effective therapies tailored to the genetic profiles of specific populations or even individual patients, thereby enhancing the precision of therapeutic interventions.
In addition to genomic analysis, proteomics plays a crucial role in understanding drug interactions at the protein level. By employing proteomic techniques, scientists can explore the dynamics of protein-protein interactions and post-translational modifications that influence drug response. This layer of complexity adds a significant dimension to drug discovery, as the identification of biomarkers related to protein activity can lead to the development of drugs that are more effective in modulating specific pathways involved in disease progression. Investigating these interactions is essential for designing drugs that not only target the intended proteins but also account for the broader network of cellular interactions.
Metabolomics, the study of small molecules and metabolites within biological systems, offers additional insights into drug efficacy and safety. By profiling metabolites, researchers can assess the biochemical changes induced by drug treatments and determine their therapeutic impact. This approach allows for the evaluation of drug responses in a holistic manner, capturing the metabolic shifts that may indicate therapeutic success or potential toxicity. As the field of metabolomics evolves, its integration with genetic and proteomic data is expected to yield a more comprehensive understanding of the multifaceted nature of drug response.
Machine learning models are increasingly being employed to streamline the drug design process, particularly in predicting responses based on biological data. These algorithms can analyze vast datasets to uncover complex patterns and relationships that may not be immediately apparent through traditional analysis. By training models on genomic, proteomic, and metabolomic data, scientists can enhance their predictive capabilities, leading to more informed decisions in the drug development process. Furthermore, integrating systems biology approaches allows for a more nuanced understanding of how drugs interact with biological systems, paving the way for the next generation of targeted therapies that are both effective and personalized.
Challenges and Opportunities in Personalized Medicine
Personalized medicine represents a significant advancement in the field of healthcare, particularly in improving therapeutic outcomes through tailored treatments based on individual patient characteristics. However, its integration into clinical practice faces several challenges. One of the primary obstacles is the complexity of genomic data analysis, which requires robust bioinformatics tools to interpret vast amounts of data generated from next-generation sequencing technologies. These tools must not only handle data processing but also ensure accuracy and reproducibility. Additionally, the interpretation of variant significance in the context of disease is fraught with difficulties, as many genetic variants remain poorly understood, complicating drug target identification.
Opportunities for personalized medicine lie in the growing availability of genomic, proteomic, and metabolomic data, which can be harnessed to enhance drug discovery and development processes. Advances in proteomics allow researchers to explore protein interactions on a systems level, leading to the identification of novel drug targets that were previously unrecognized. Similarly, metabolomics offers insights into the biochemical alterations associated with disease states, enabling the assessment of drug efficacy and safety profiles in personalized treatment paradigms. By leveraging these omics technologies, scientists can create a more comprehensive understanding of disease mechanisms, ultimately leading to targeted therapies that are more effective for specific patient populations.
The integration of machine learning models in drug design further amplifies the potential of personalized medicine. By utilizing algorithms trained on biological data, researchers can predict the effectiveness and safety of new drug molecules with greater precision. This predictive power is particularly beneficial in early-stage drug discovery, where computational methods can significantly reduce the time and resources needed to identify viable drug candidates. Machine learning can also facilitate the identification of biomarkers that correlate with patient responses to therapies, enabling a more tailored approach to treatment selection and optimization.
Despite these opportunities, challenges in data integration and clinical application persist. The need for comprehensive clinical data integration is paramount for the successful translation of personalized medicine into practice. This includes not only genomic data but also clinical trial outcomes and patient demographics, which must be harmonized to provide actionable insights. Additionally, regulatory frameworks need to adapt to accommodate the nuances of personalized therapies, ensuring that safety and efficacy are evaluated in the context of diverse patient populations. Addressing these challenges will require collaboration among bioinformaticians, clinicians, and regulatory bodies to create a streamlined pathway for the implementation of personalized medicine.
Ultimately, the interplay of challenges and opportunities in personalized medicine underscores the importance of bioinformatics in the drug discovery process. By optimizing lead compounds through structural bioinformatics, utilizing advanced computational models, and integrating multi-omics data, scientists can enhance the precision of targeted therapies. As the field continues to evolve, the potential for personalized medicine to revolutionize healthcare becomes increasingly tangible, promising not only to improve patient outcomes but also to pave the way for a more efficient and effective drug development landscape.
Chapter 11: Clinical Data Integration for Drug Development
Importance of Clinical Data in Drug Discovery
The role of clinical data in drug discovery is paramount, as it serves as the bridge between preclinical research and the eventual development of effective therapeutics. In the realm of bioinformatics, the integration of clinical data enhances the understanding of disease mechanisms, patient variability, and treatment outcomes. This information is crucial for identifying potential drug targets and refining lead compounds, ultimately guiding the decision-making process in drug development. The incorporation of clinical data ensures that the findings from genomic, proteomic, and metabolomic studies are translated into actionable insights that can lead to successful therapies.
Genomic data analysis plays a vital role in the identification of drug targets by revealing genetic mutations and aberrations associated with various diseases. By integrating clinical data with genomic information, researchers can pinpoint specific genes or pathways that are implicated in disease progression. This targeted approach not only facilitates the discovery of novel drug targets but also helps in understanding patient heterogeneity, allowing for the development of personalized medicine strategies. The ability to correlate clinical outcomes with genomic profiles is essential for validating the efficacy of potential drug candidates and for designing clinical trials that are more likely to yield significant results.
Proteomics, the study of proteins and their interactions, provides another layer of insight into drug discovery. Clinical data can enhance proteomic analysis by offering context regarding which proteins are expressed in specific diseases and how they interact with drug candidates. Understanding these interactions is critical for optimizing lead compounds, as it allows scientists to identify which modifications may improve binding affinity and specificity. Furthermore, proteomic profiling can reveal biomarkers that predict treatment response, thereby aiding in patient stratification for clinical trials and improving the chances of successful therapeutic outcomes.
Metabolomics also plays a crucial role in assessing drug efficacy and safety. By analyzing metabolic profiles in clinical samples, researchers can evaluate how a drug candidate affects metabolic pathways and whether it leads to beneficial or adverse effects. The integration of clinical data in metabolomic studies allows for a more comprehensive understanding of drug action in the human body. This knowledge can guide the optimization of lead compounds by identifying metabolites that correlate with therapeutic efficacy or toxicity, thus refining the drug development process.
Finally, the application of machine learning models in drug design has revolutionized the way scientists approach drug discovery. By leveraging clinical data alongside biological datasets, machine learning algorithms can identify patterns and predict the effectiveness of new drug molecules. This predictive capability accelerates the screening of potential drug candidates and enhances the prioritization of compounds for further development. Additionally, the integration of clinical trial data with preclinical findings through systems biology approaches allows for a holistic view of drug interactions within complex biological systems. Such comprehensive data utilization not only streamlines the drug development process but also fosters innovation in creating targeted therapies that meet the needs of diverse patient populations.
Integrating Preclinical and Clinical Data
Integrating preclinical and clinical data represents a critical aspect of modern drug discovery and development. As the landscape of biopharmaceutical research evolves, the synergy between preclinical findings and clinical trial outcomes has become increasingly important. This integration not only enhances the understanding of how drug candidates behave in biological systems but also informs decisions regarding their efficacy, safety, and suitability for specific patient populations. By bridging the gap between these two phases of drug development, scientists can optimize lead compounds and streamline the path from laboratory research to clinical application.
One of the key areas where this integration is vital is in genomic data analysis for drug target identification. The identification of potential drug targets often begins with genomic studies that reveal genetic variations associated with disease states. By correlating preclinical genomic findings with clinical data, researchers can validate these targets and assess their relevance in human populations. This approach ensures that drug candidates are not only effective in vitro but also hold promise for translating into clinical efficacy. It allows for a more informed selection of targets that are likely to yield successful therapeutic outcomes.
Proteomics plays an equally important role in integrating preclinical and clinical data, particularly in understanding protein interactions and their implications in drug development. High-throughput proteomic techniques enable the analysis of protein expression and interaction networks in both preclinical models and clinical samples. By comparing these datasets, scientists can identify biomarkers of drug response and resistance, providing insights into how different patients may respond to a given treatment. This information is crucial for refining drug candidates and tailoring therapies to enhance patient outcomes.
Metabolomics, the study of metabolic profiles, further complements the integration of preclinical and clinical data by evaluating the efficacy and safety of new drug candidates. Preclinical studies often generate metabolomic data that highlight how a drug alters metabolic pathways in model organisms. When this data is integrated with clinical metabolomic profiles, researchers can assess whether the anticipated metabolic changes occur in humans. This alignment between preclinical and clinical findings not only helps in predicting drug efficacy but also in identifying potential safety concerns early in the development process.
Finally, the incorporation of machine learning models in drug design significantly enhances the integration of preclinical and clinical data. By training algorithms on extensive biological datasets, researchers can predict the effectiveness of new drug molecules based on historical data from both preclinical and clinical studies. These models can identify patterns that may not be readily apparent through traditional analysis, ultimately leading to more informed decision-making in drug development. In addition, virtual screening techniques and bioinformatics tools for drug repurposing can be employed to rapidly evaluate large compound libraries, ensuring that the most promising candidates are selected for further development based on a thorough understanding of both preclinical and clinical contexts. This integrated approach is essential for advancing personalized medicine, as it allows for the customization of therapeutic strategies based on individual genetic profiles and responses.
Enhancing Drug Development through Data Integration
Data integration has emerged as a pivotal strategy in enhancing drug development, particularly in the context of structural bioinformatics. By amalgamating diverse datasets from genomic, proteomic, and metabolomic sources, researchers can gain comprehensive insights into the biological underpinnings of diseases and drug responses. This multifaceted approach not only accelerates the identification of potential drug targets but also optimizes lead compounds, ultimately leading to more effective and personalized therapies. As the complexity of biological systems continues to be unraveled, the integration of various data types becomes essential for advancing drug discovery and development.
Genomic data analysis plays a crucial role in identifying new drug targets by revealing the genetic variations associated with specific diseases. By integrating genomic datasets with clinical data, scientists can pinpoint mutations and expression changes that may serve as actionable targets in therapeutic development. This data-driven identification process is further enhanced when combined with machine learning models, which can predict the likelihood of success for newly identified targets based on historical data. The synergy between genomics and machine learning not only streamlines the target identification process but also facilitates the prioritization of candidates for further investigation.
Proteomics, which focuses on the large-scale study of proteins, adds another layer of depth to drug development. By utilizing proteomic techniques, researchers can elucidate protein-protein interactions and signaling pathways involved in disease progression. Integrating proteomic data with genomic information allows for a more holistic view of the cellular environment, which is crucial for understanding how drugs interact with their targets. This integration helps in refining lead compounds by providing insights into off-target effects and enhancing binding affinity, thus ensuring that the most promising candidates are selected for further development.
Metabolomics, the study of metabolites within biological systems, also plays an integral role in assessing drug efficacy and safety. By profiling metabolic changes induced by drug candidates, researchers can evaluate their pharmacodynamic properties and potential side effects. Integrating metabolomic data with clinical trial results offers a feedback loop that informs the optimization of lead compounds. This comprehensive assessment not only aids in the identification of biomarkers for patient stratification but also enhances the overall understanding of drug behavior in vivo.
Finally, the integration of clinical data with preclinical findings is essential for bridging the gap between laboratory research and clinical application. By leveraging bioinformatics tools to analyze data from diverse sources, scientists can make informed decisions that enhance the drug development process. This systematic approach fosters collaboration among different disciplines, supporting the design of more effective clinical trials and facilitating the transition of promising drug candidates from bench to bedside. As the field of structural bioinformatics continues to evolve, the importance of data integration in enhancing drug development cannot be overstated, paving the way for innovative therapies that are more tailored and effective.
Future Perspectives on Clinical Data Utilization
The future perspectives on clinical data utilization within the realm of structural bioinformatics promise to significantly enhance the landscape of drug discovery and development. As the amount of available clinical data continues to grow, the integration of this information with genomic, proteomic, and metabolomic analyses will become increasingly vital. Advances in technologies that facilitate the collection and analysis of clinical data will enable researchers to glean insights that were previously unattainable. This evolution will allow for more precise identification of drug targets, fostering the development of therapies tailored to specific patient populations and disease profiles.
A key area of focus will be genomic data analysis, which holds immense potential for drug target identification. By leveraging large-scale genomic datasets, researchers can uncover genetic variations that contribute to disease pathology, thus identifying novel targets for intervention. The integration of clinical data with genomic profiling will facilitate a deeper understanding of how these variations influence drug response, ultimately guiding the selection of candidates for clinical trials. This synergy between genomic insights and clinical outcomes will not only streamline the drug development process but also enhance the likelihood of success in targeting complex diseases.
Proteomics, another critical component of drug discovery, will benefit greatly from advancements in clinical data utilization. By applying proteomic techniques, scientists can elucidate protein interactions and their roles in disease mechanisms. The integration of clinical data will allow for the identification of biomarkers that indicate therapeutic efficacy or toxicity, providing a more comprehensive picture of how drug candidates interact within biological systems. This knowledge will be pivotal in refining lead compounds and optimizing their pharmacological properties, ensuring that new therapies are both effective and safe for patients.
The rise of machine learning models in drug design is set to revolutionize how clinical data is utilized. By developing sophisticated algorithms that analyze biological data, researchers can predict the effectiveness of new drug molecules with impressive accuracy. These models can integrate diverse datasets, including clinical trial results, to uncover patterns that inform drug development strategies. As machine learning continues to evolve, its application in predicting patient responses and personalizing therapies will become increasingly refined, driving the future of tailored medicine.
Finally, the integration of clinical data with systems biology approaches will enhance our understanding of the complex interactions within biological systems during drug discovery. This holistic view will allow researchers to assess how various factors—genomic, proteomic, and metabolomic—interact in the context of drug efficacy and safety. As bioinformatics tools become more sophisticated, the ability to conduct virtual screening of compound libraries will also be enhanced, allowing for rapid identification of potential drug candidates. Together, these advancements will pave the way for a more efficient and effective drug development process, ultimately leading to breakthroughs in personalized medicine and improved patient outcomes.
Chapter 12: Conclusion and Future Directions
Summary of Key Concepts
The subchapter "Summary of Key Concepts" encapsulates the foundational elements that bridge structural bioinformatics and targeted therapy optimization. In the realm of bioinformatics technology for new drug discovery, the integration of genomic data analysis stands out as a critical component. Scientists leverage genomic data to identify potential drug targets, unraveling the genetic underpinnings of various diseases. By pinpointing specific gene mutations and pathways associated with disease progression, researchers can focus their efforts on developing targeted therapies that address the root causes of illnesses rather than merely treating symptoms. This genomic-centric approach enhances the precision of drug discovery, ensuring that new treatments are both effective and personalized.
Proteomics plays an equally vital role in drug discovery by elucidating protein interactions and functions. The application of proteomic techniques enables scientists to analyze the dynamic landscape of protein expression, modifications, and interactions within a biological system. Understanding how proteins interact with potential drug molecules is essential for predicting therapeutic efficacy and minimizing adverse effects. By integrating proteomic data with genomic insights, researchers can develop a more comprehensive understanding of disease mechanisms, paving the way for innovative therapeutic strategies.
Metabolomics, the study of small molecules and metabolites within biological systems, further enriches the drug development process. By utilizing metabolomic profiling, scientists can assess the efficacy and safety of new drug candidates. This approach allows for the identification of biomarkers that indicate a drug's pharmacological effects and potential toxicity. The holistic view provided by metabolomics complements genomic and proteomic data, enabling a multidimensional analysis of how drugs influence biological pathways. As a result, the drug development pipeline benefits from a more thorough evaluation of candidate compounds, ultimately leading to safer and more effective therapies.
Machine learning models are revolutionizing drug design by harnessing vast amounts of biological data to predict the effectiveness of new drug molecules. By employing sophisticated algorithms, researchers can analyze complex datasets and identify patterns that inform drug design decisions. This computational approach not only accelerates the discovery process but also enhances the likelihood of success in developing new therapeutics. The synergy between structural bioinformatics and machine learning empowers scientists to optimize lead compounds for improved binding affinity and specificity, ensuring that drug candidates meet the desired therapeutic criteria.
Lastly, the incorporation of systems biology approaches in pharmacology epitomizes the trend towards understanding the intricate interactions within biological systems during drug discovery. By viewing biological processes as interconnected networks rather than isolated pathways, scientists can better comprehend the multifactorial nature of diseases. Virtual screening techniques further streamline the identification of potential drug candidates by allowing researchers to evaluate extensive compound libraries rapidly. Together, these methodologies highlight the transformative impact of bioinformatics in drug discovery, encompassing everything from drug repurposing to personalized medicine. The integration of clinical data with preclinical findings enhances the overall drug development process, ensuring that innovative therapies are both effective and tailored to meet the unique needs of patients.
Emerging Trends in Structural Bioinformatics
Emerging trends in structural bioinformatics are reshaping the landscape of drug discovery and development, offering innovative methodologies that enhance the identification and optimization of lead compounds. As the field continues to evolve, integrating genomic data analysis has become paramount for identifying potential drug targets for a variety of diseases. By leveraging high-throughput sequencing technologies, researchers can uncover mutations and expression profiles that indicate viable therapeutic targets. This trend not only accelerates the drug discovery pipeline but also enables a more personalized approach to medicine, as genomic insights can help tailor therapies to specific patient populations based on their unique genetic makeup.
Another significant trend is the application of proteomics in drug discovery. Proteomics techniques, such as mass spectrometry and protein interaction mapping, provide a comprehensive view of protein dynamics and interactions within biological systems. Understanding these interactions is crucial for identifying how drug candidates might affect cellular pathways and for determining the potential off-target effects of compounds. By integrating proteomics with structural bioinformatics, scientists can gain insights into the three-dimensional structures of target proteins, facilitating the design of more effective and selective small molecules that can modulate these targets.
Metabolomics is also emerging as a vital component in assessing drug efficacy and safety. By profiling metabolites in biological samples, researchers can evaluate the biochemical impacts of drug candidates at various stages of development. This trend allows for a more holistic understanding of how drugs influence metabolic pathways, helping to identify biomarkers of efficacy or toxicity early in the development process. Such insights not only optimize lead compounds but also inform clinical decision-making, paving the way for more robust therapeutic interventions.
The incorporation of machine learning models into drug design is revolutionizing the predictive capabilities of bioinformatics. Advanced algorithms can analyze vast datasets derived from genomic, proteomic, and metabolomic studies to identify patterns and predict the effectiveness of new drug molecules. This trend enhances the ability to prioritize compounds for further development based on their predicted binding affinities and biological activity, significantly reducing the time and resources needed for experimental validation. As machine learning techniques become increasingly sophisticated, their integration with structural bioinformatics will likely yield even more accurate predictions, streamlining the drug discovery process.
Finally, the integration of systems biology approaches in pharmacology underscores the complexity of biological systems and the interactions that govern drug action. By adopting a holistic view of cellular networks, researchers can better understand the multifaceted effects of drug candidates. Coupled with virtual screening techniques, which allow for the rapid evaluation of large compound libraries, this systems biology perspective facilitates the identification of promising drug candidates that might otherwise be overlooked. As these emerging trends converge, they promise to enhance the efficacy of drug discovery efforts, leading to more effective therapies tailored to the intricate dynamics of human biology.
The Future of Drug Discovery and Development
The future of drug discovery and development is poised for transformative changes driven by advancements in bioinformatics and related technologies. As the complexity of biological systems becomes increasingly understood, the integration of genomic, proteomic, and metabolomic data will play a pivotal role in identifying and validating new drug targets. Genomic data analysis, in particular, provides insights into genetic variations that influence disease mechanisms, allowing researchers to pinpoint potential drug targets with greater precision. By harnessing large-scale sequencing data and employing sophisticated analytical techniques, scientists can uncover the genetic underpinnings of various diseases, paving the way for targeted therapies that are more effective and tailored to patient profiles.
Proteomics, the study of protein structures and interactions, is another cornerstone in the future landscape of drug discovery. The application of proteomic techniques enables researchers to explore the dynamic interactions between proteins and their roles in cellular processes. Understanding these interactions is crucial for drug development, as it can reveal key pathways that are altered in disease states. By utilizing advanced proteomic technologies, scientists can identify biomarkers for disease progression and drug response, enhancing the ability to design drugs that are not only effective but also have minimal side effects. This approach fosters a more comprehensive understanding of the molecular mechanisms that underpin diseases, ultimately leading to the design of more targeted and efficient therapeutic interventions.
Metabolomics, the study of metabolic profiles, is emerging as a vital component of drug efficacy assessment. By profiling metabolites in biological samples, researchers can gain insights into the biochemical effects of drug candidates and their mechanisms of action. This information is invaluable for assessing the safety and efficacy of new drug molecules, as it provides a holistic view of how drugs interact with biological systems. The integration of metabolomic data with other omics technologies will allow for a more nuanced understanding of the pharmacodynamics and pharmacokinetics of drug candidates, facilitating the identification of compounds that not only target disease but also promote overall metabolic balance.
The integration of machine learning into drug design represents a significant leap forward in the efficiency and effectiveness of drug discovery processes. By developing algorithms that analyze vast datasets, researchers can predict the biological activity of new drug molecules based on their chemical structures and biological properties. Machine learning models can identify patterns and correlations that might be overlooked in traditional approaches, enabling the rapid evaluation of potential drug candidates. This predictive capability is particularly useful in optimizing lead compounds, allowing for iterative refinement processes that enhance binding affinity and specificity, thus improving the likelihood of clinical success.
As we look towards the future, the incorporation of systems biology approaches into pharmacology will further enhance our understanding of drug interactions within complex biological networks. By integrating data from various biological levels—genomic, proteomic, and metabolomic—systems biology offers a comprehensive framework for exploring the multifaceted nature of disease and drug response. Additionally, the application of virtual screening techniques will streamline the exploration of extensive compound libraries, enabling researchers to identify promising candidates more efficiently. Coupled with bioinformatics tools for drug repurposing and the growing emphasis on personalized medicine, the future of drug discovery and development holds great promise for creating innovative therapies that are tailored to the unique genetic and biochemical landscapes of individual patients.
Final Thoughts on the Role of Bioinformatics in Medicine
The integration of bioinformatics into the field of medicine has proven to be a transformative force, particularly in drug discovery and development. As we have explored throughout this book, bioinformatics serves as a crucial bridge between biological data and therapeutic applications. By harnessing the power of genomic data analysis, researchers can identify potential drug targets for various diseases, paving the way for more effective and targeted therapies. The ability to analyze vast amounts of genomic information allows scientists to pinpoint specific mutations and molecular pathways that can be exploited for therapeutic intervention, emphasizing the role of bioinformatics in the evolving landscape of personalized medicine.
Additionally, proteomics plays a vital role in understanding the complex interactions of proteins within biological systems. By employing proteomic techniques, researchers can assess protein expressions and interactions, which are essential for elucidating mechanisms of action for new drug candidates. This information is invaluable for optimizing lead compounds, as understanding protein dynamics can guide modifications that enhance binding affinity and specificity. The synergy between proteomics and structural bioinformatics enables the refinement of drug candidates, ensuring that therapies not only target the intended proteins but also navigate the intricate web of cellular interactions effectively.
Metabolomics further complements the bioinformatics landscape by providing insights into the efficacy and safety of drug candidates. By profiling metabolites, researchers can evaluate how drugs affect metabolic pathways and identify potential biomarkers for therapeutic response. This holistic approach enhances the understanding of drug mechanisms and helps assess the long-term impacts of therapies. In conjunction with machine learning models, which predict drug efficacy based on biological data, metabolomic profiling can streamline the drug development process, allowing for more informed decision-making and resource allocation.
The application of systems biology approaches in pharmacology underscores the importance of viewing drug discovery through a comprehensive lens. By integrating various biological data types—genomic, proteomic, and metabolomic—scientists can construct a more complete picture of how drugs interact with complex biological systems. This systems-level understanding is critical for identifying unforeseen interactions and optimizing therapeutic strategies. Furthermore, virtual screening techniques enable the rapid exploration of large compound libraries, identifying promising candidates for further investigation, thus expediting the initial phases of drug discovery.
In conclusion, bioinformatics has emerged as an indispensable tool in modern medicine, facilitating the discovery and development of novel therapeutics. As we continue to harness advancements in technology and data analysis, the potential for bioinformatics to enhance personalized medicine, improve drug repurposing strategies, and streamline clinical data integration will only grow. The collaborative efforts between bioinformatics and various scientific domains promise to yield innovative solutions, ultimately improving patient outcomes and ushering in a new era of targeted therapies. The ongoing evolution of bioinformatics in medicine not only reflects the dynamic nature of scientific inquiry but also highlights the potential for transformative advancements in healthcare.